5
1
9783030378295
Implementations and Applications of Machine Learning available in Hardcover, eBook
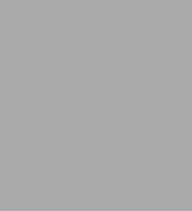
Implementations and Applications of Machine Learning
- ISBN-10:
- 3030378292
- ISBN-13:
- 9783030378295
- Pub. Date:
- 04/24/2020
- Publisher:
- Springer International Publishing
- ISBN-10:
- 3030378292
- ISBN-13:
- 9783030378295
- Pub. Date:
- 04/24/2020
- Publisher:
- Springer International Publishing
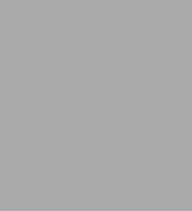
Implementations and Applications of Machine Learning
$169.99
Current price is , Original price is $169.99. You
169.99
In Stock
Product Details
ISBN-13: | 9783030378295 |
---|---|
Publisher: | Springer International Publishing |
Publication date: | 04/24/2020 |
Series: | Studies in Computational Intelligence , #782 |
Edition description: | 1st ed. 2020 |
Pages: | 280 |
Product dimensions: | 6.10(w) x 9.25(h) x (d) |
About the Author
From the B&N Reads Blog