5
1
9789813209008
Econometric Models For Industrial Organization available in Hardcover, Paperback, eBook
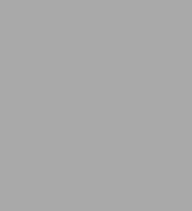
Econometric Models For Industrial Organization
- ISBN-10:
- 9813209003
- ISBN-13:
- 9789813209008
- Pub. Date:
- 02/03/2017
- Publisher:
- World Scientific Publishing Company, Incorporated
- ISBN-10:
- 9813209003
- ISBN-13:
- 9789813209008
- Pub. Date:
- 02/03/2017
- Publisher:
- World Scientific Publishing Company, Incorporated
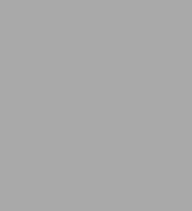
Econometric Models For Industrial Organization
$40.0
Current price is , Original price is $40.0. You
40.0
In Stock
Product Details
ISBN-13: | 9789813209008 |
---|---|
Publisher: | World Scientific Publishing Company, Incorporated |
Publication date: | 02/03/2017 |
Series: | World Scientific Lecture Notes In Economics , #3 |
Pages: | 156 |
Product dimensions: | 5.90(w) x 8.90(h) x 0.40(d) |
From the B&N Reads Blog