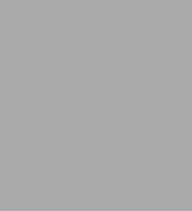
The Practice of Reproducible Research: Case Studies and Lessons from the Data-Intensive Sciences
368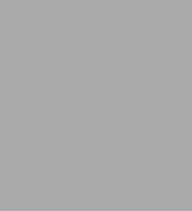
The Practice of Reproducible Research: Case Studies and Lessons from the Data-Intensive Sciences
368eBook
Available on Compatible NOOK devices, the free NOOK App and in My Digital Library.
Related collections and offers
Overview
Part 1 provides an accessible introduction to reproducible research, a basic reproducible research project template, and a synthesis of lessons learned from across the thirty-one case studies. Parts 2 and 3 focus on the case studies themselves. The Practice of Reproducible Research is an invaluable resource for students and researchers who wish to better understand the practice of data-intensive sciences and learn how to make their own research more reproducible.
Product Details
ISBN-13: | 9780520967779 |
---|---|
Publisher: | University of California Press |
Publication date: | 10/17/2017 |
Sold by: | Barnes & Noble |
Format: | eBook |
Pages: | 368 |
File size: | 7 MB |
About the Author
Daniel Turek is Assistant Professor of Statistics at Williams College.
Fatma Deniz is Postdoctoral Scholar at the Helen Wills Neuroscience Institute and the International Computer Science Institute, and Data Science Fellow at the University of California, Berkeley.
Read an Excerpt
CHAPTER 1
Assessing Reproducibility
ARIEL ROKEM, BEN MARWICK, AND VALENTINA STANEVA
While understanding the full complement of factors that contribute to reproducibility is important, it can also be hard to break down these factors into steps that can immediately be adopted into an existing research program and immediately improve its reproducibility. One of the first steps to take is to assess the current state of affairs, and to track improvement as steps are taken to increase reproducibility even more. This chapter provides a few key points for this assessment.
WHAT IT MEANS TO MAKE RESEARCH REPRODUCIBLE
Although one of the objectives of this book is to discover how researchers are defining and implementing reproducibility for themselves, it is important at this point to briefly review some of the current scholarly discussion on what it means to strive for reproducible research. This is important because recent surveys and commentary have highlighted that there is confusion among scientists about the meaning of reproducibility (Baker, 2016a, 2016b). Furthermore, there is disagreement about how to define "reproducible" and "replicable" in different fields (Drummond, 2009; Casadevall & Fang, 2010; Stodden et al., 2013; Easterbrook, 2014). For example, Goodman et al. (2016) note that in epidemiology, computational biology, economics, and clinical trials, reproducibility is often defined as:
the ability of a researcher to duplicate the results of a prior study using the same materials as were used by the original investigator. That is, a second researcher might use the same raw data to build the same analysis files and implement the same statistical analysis in an attempt to yield the same results.
This is distinct from replicability:
which refers to the ability of a researcher to duplicate the results of a prior study if the same procedures are followed but new data are collected.
It is noteworthy that definitions above, which are broadly consistent with usage of these terms throughout this book, are totally opposite to the Association for Computing Machinery (ACM, the world's largest scientific computing society), which take their definitions from the International Vocabulary of Metrology. Here are the ACM definitions:
Reproducibility (Different team, different experimental setup) The measurement can be obtained with stated precision by a different team, a different measuring system, in a different location on multiple trials. For computational experiments, this means that an independent group can obtain the same result using artifacts which they develop completely independently.
Replicability (Different team, same experimental setup) The measurement can be obtained with stated precision by a different team using the same measurement procedure, the same measuring system, under the same operating conditions, in the same or a different location on multiple trials. For computational experiments, this means that an independent group can obtain the same result using the author's own artifacts.
We can see the heritage of the definitions of the ACM in literature on physics and the philosophy of science (Collins, 1984; Franklin & Howson, 1984; Cartwright, 1991). In her paper on the epistemology of scientific experimentation, Cartwright (1991) presents one of the first clear definitions of the key terms: "replicability — doing the same experiment again" and "reproducibility — doing a new experiment."
The definition of Cartwright is at odd with our preferred definition, from Goodman et al. (2016). This is because we trace a different ancestry in the use of the term "reproducible," one that recognizes the central role of the computer in scientific practice, with less emphasis on empirical experimentation as the primary means for generating knowledge. Among the first to write about reproducibility in this way is geophysicist Jon Claerbout. He pioneered the use of the phrase "reproducible research" to describe how his seismology research group used computer programs to enable efficient regeneration of the figures and tables in theses and publications (Claerbout & Karrenfach, 1992). We can see this usage more recently in Stodden et al. (2014):
Replication, the practice of independently implementing scientific experiments to validate specific findings, is the cornerstone of discovering scientific truth. Related to replication is reproducibility, which is the calculation of quantitative scientific results by independent scientists using the original data sets and methods. Reproducibility can be thought of as a different standard of validity because it forgoes independent data collection and uses the methods and data collected by the original investigator. Reproducibility has become an important issue for more recent research due to advances in technology and the rapid spread of computational methods across the research landscape.
It is this way of thinking about reproducibility that captures most of the variation in the way the contributors to this book use the term. One of the key ideas that the remainder of this chapter explores is that reproducibility is a matter of degree, rather than kind. Identifying the factors that can relatively easily and quickly be changed can incrementally lead to an increase in the reproducibility of a research program. Identifying more challenging points, that would require more work, helps set long-term goals toward even more reproducible work, and helps identify practical changes that can be made over time.
Reproducibility can be assessed at several different levels: at the level of an individual project (e.g., a paper, an experiment, a method, or a data set), an individual researcher, a lab or research group, an institution, or even a research field. Slightly different kinds of criteria and points of assessment might apply to these different levels. For example, an institution upholds reproducibility practices if it institutes policies that reward researchers who conduct reproducible research. Meanwhile, a research field might be considered to have a higher level of reproducibility if it develops community-maintained resources that promote and enable reproducible research practices, such as data repositories, or common data-sharing standards.
This book focuses on the first of these levels, that of a specific research project. In this chapter we consider some of the ways that reproducibility can be assessed by researchers who might be curious about how they can improve their work. We have divided this assessment of reproducibility into three different broad aspects: automation and provenance tracking, availability of software and data, and open reporting of results. For each aspect we provide a set of questions to focus attention on key details where reproducibility can be enhanced. In some cases we provide specific suggestions about how the questions could be answered, where we think the suggestions might be useful across many fields.
The diversity of standards and tools relating to reproducible research is large and we cannot survey all the possible options in this chapter. We recommend that researchers use the detailed case studies in following chapters for inspiration, tailoring choices to the norms and standards of their discipline.
AUTOMATION AND PROVENANCE TRACKING
Automation of the research process means that the main steps in the project: transformations of the data — various processing steps and calculations — as well as the visualization steps that lead to the important inferences, are encoded in software and documented in such a way that they can reliably and mechanically be replicated. In other words, the conclusions and illustrations that appear in the article are the result of a set of computational routines, or scripts that can be examined by others, and rerun to reproduce these results.
To assess the sufficiency of automation in a project, one might ask:
Can all figures/calculations that are important for the inference leading to the result be reproduced in a single button press? If not a single button press, can these be produced with a reasonably small effort? One way to achieve this goal is to write software scripts that embody every step in the analysis up to the creation of figures, and derivation of calculations. In assessment, you can ask: is it possible to point to the software script (or scripts) that generated every one of the calculations and data visualizations? Is it possible to run these scripts with reasonably minimal effort?
Another set of questions refers to the starting point of the calculations in the previous question: what is the starting point of running these scripts? What is required as setup steps to the calculations in these scripts? If the setup includes manual processing of data, or cumbersome setup of a computational environment, this detracts from the reproducibility of the research.
The main question underlying these criteria is how difficult it would be for another researcher to first reproduce the results of a research project, and then further build upon these results. Because research is hard, and errors are ubiquitous (a point made in this context by Donoho et al., 2008), the first person to benefit from automation is often the researcher performing the original research, when hunting down and eliminating errors.
Provenance tracking is very closely related to automation (see glossary for definitions). It entails that the full chain of computational events that occurred from the raw data to a conclusion is tracked and documented. In cases in which automation is implemented, provenance tracking can be instantiated and executed with a reasonably minimal effort.
When large data sets and complex analysis are involved, some processing steps may consume more time and computational resources than can be reasonably required to be repeatedly executed. In these cases, some other form of provenance tracking may serve to bolster reproducibility, even in the absence of a fully automatic processing pipeline. Items for assessment here are:
If software was used in (pre)processing the data, is this software properly described? This includes documentation of the version of the software that was used, and the settings of parameters that were used as inputs to this software.
If databases were queried, are the queries fully documented? Are dates of access recorded?
Are scripts for data cleaning included with the research materials, and do they include commentary to explain key decisions made about missing data and discarding data?
Another aspect of provenance tracking is the tracking of different versions of the software, and recording of the evolution of the software, including a clear delineation of the versions of the software that were used to support specific scientific findings. This can be assessed by asking: Is the evolution of the software available for inspection through a publicly accessible version control system? Are versions that contributed to particular findings clearly tagged in the version control history?
AVAILABILITY OF DATA AND SOFTWARE
The public availability of the data and software are key components of computational reproducibility. To facilitate its evaluation, we suggest that researchers consider the following series of questions.
Availability of Data
Are the data available through an openly accessible database? Often data is shared through the Internet. Here, we might ask about the long-term reliability of the Web address: are the URLs mentioned in a manuscript permanently and reliably assigned to the data set? One example of a persistent URL is a Digital Object Identifier (DOI). Several major repositories provide these for data sets (e.g., Figshare). Data sets accessible via persistent URLs increase the reproducibility of the research, relative to use of an individually maintained website, such as a lab group website or a researcher's personal website. This is because when an individually maintained websites changes its address or structure over time, the previously published URLs may no longer work. In many academic institutions, data repositories that provide persistent URLs are maintained by the libraries. These data repositories provide a secure environment for long-term citation, access, and reuse of research data.
Are the data shared in a commonly used and well-documented file format? For tabular data, open file formats based on plain text, such as CSV (comma separated values) or TSV (tab separated values) are often used. The main benefit of text-based formats is their simplicity and transparency. On the other hand, they suffer from a loss of numerical precision, they are relatively large, and parsing them might still be difficult. Where available, strongly typed binary formats should be preferred. For example multidimensional array data can be stored in formats such as HDF5. In addition, there are also open data formats that have been developed in specific research communities to properly store data and metadata relevant to the analysis of data from this research domain. Examples include the FITS data format for astronomical data (Wells et al., 1981), and the NIFTI and DICOM file formats for medical imaging data (Larobina & Murino, 2014).
Proprietary file formats are problematic for reproducibility because they may not be usable on future computer systems due to intellectual property restrictions, obsolescence or incompatibility. However, one can still ask: if open formats are not suitable, is software provided to read the data into computer memory with reasonably minimal effort?
If community standards exist, are files laid out in the shared database in a manner that conforms with these standards? For example, for neuroimaging data, does the file layout follow the Brain Imaging Data Structure (Gorgolewski et al., 2016) format?
If data are updated, are different versions of the data clearly denoted? If data is processed in your analysis, is the raw data available?
Is sufficient metadata provided? The type and amount of metadata varies widely by area of research, but a minimal set might include the research title, authors' names, description of collection methods and measurement variables, date, and license.
If the data are not directly available, for example if the data are too large to share conveniently, or have restrictions related to privacy issues, do you provide sufficient instructions to obtain equivalent data? For example, are the experimental protocols used to acquire the original data sufficiently detailed?
Availability of Software
Is the software available to download and install? Software can also be deposited in repositories that issue persistent URLs, just like data sets. This can improve the longevity of its accessibility.
Can the software easily be installed on different platforms? If a scripting language such as Python or R was used, it is better for reproducibility to share the source rather than compiled binaries that are platform-specific.
Does the software have conditions on the use? For example, license fees, restrictions to academic or noncommercial use, etc.
Is the source code available for inspection?
Is the full history of the source code available for inspection through a publicly available version history?
Are the dependencies of the software (hardware and software) described properly? Do these dependencies require only a reasonably minimal amount of effort to obtain and use? For example, if a research project requires the use of specialized hardware, it will be harder to reproduce. If it depends on expensive commercial software, likewise. Use of open-source software dependencies on commodity hardware is not always possible, but when possible electing to use these increases reproducibility.
Software Documentation
Documentation of the software is another factor in removing barriers to reuse. Several forms of documentation can be added to a research repository and each of them adds to reproducibility. Relevant questions include:
Does the software include a README file? This provides information about the purpose of the software, its use and ways to contact the authors of the software (see more below).
Is there any function/module documentation? This closely explains the different parts of the code, including the structure of the modules that make up the code; the inputs and outputs of functions; the methods and attributes of objects, etc.
Is there any narrative documentation? This explains how the bits and pieces of the software work together; narrative documentation might also explain how the software should be installed and configured in different circumstances and can explain in what order things should be executed.
(Continues…)
Excerpted from "The Practice of Reproducible Research"
by .
Copyright © 2018 The Regents of the University of California.
Excerpted by permission of UNIVERSITY OF CALIFORNIA PRESS.
All rights reserved. No part of this excerpt may be reproduced or reprinted without permission in writing from the publisher.
Excerpts are provided by Dial-A-Book Inc. solely for the personal use of visitors to this web site.
Table of Contents
ContributorsPreface: Nullius in Verba
Philip B. Stark
Introduction
Justin Kitzes
PART I: PRACTICING REPRODUCIBILITY
Assessing Reproducibility
Ariel Rokem, Ben Marwick, and Valentina Staneva
The Basic Reproducible Workflow Template
Justin Kitzes
Case Studies in Reproducible Research
Daniel Turek and Fatma Deniz
Lessons Learned
Kathryn Huff
Building toward a Future Where Reproducible, Open Science Is the Norm
Karthik Ram and Ben Marwick
Glossary
Ariel Rokem and Fernando Chirigati
PART II: HIGH-LEVEL CASE STUDIES
Case Study 1: Processing of Airborne Laser Altimetry Data Using Cloud-Based Python and Relational Database Tools
Anthony Arendt, Christian Kienholz, Christopher Larsen, Justin Rich, and Evan Burgess
Case Study 2: The Trade-Off between Reproducibility and Privacy in the Use of Social Media Data to Study Political Behavior
Pablo Barberá
Case Study 3: A Reproducible R Notebook Using Docker
Carl Boettiger
Case Study 4: Estimating the Effect of Soldier Deaths on the Military Labor Supply
Garret Christensen
Case Study 5: Turning Simulations of Quantum Many- Body Systems into a Provenance-Rich Publication
Jan Gukelberger and Matthias Troyer
Case Study 6: Validating Statistical Methods to Detect Data Fabrication
Chris Hartgerink
Case Study 7: Feature Extraction and Data Wrangling for Predictive Models of the Brain in Python
Chris Holdgraf
Case Study 8: Using Observational Data and Numerical Modeling to Make Scientific Discoveries in Climate Science
David Holland and Denise Holland
Case Study 9: Analyzing Bat Distributions in a Human- Dominated Landscape with Autonomous Acoustic Detectors and Machine Learning Models
Justin Kitzes
Case Study 10: An Analysis of Household Location Choice in Major US Metropolitan Areas Using R
Andy Krause and Hossein Estiri
Case Study 11: Analyzing Cosponsorship Data to Detect Networking Patterns in Peruvian Legislators
José Manuel Magallanes
Case Study 12: Using R and Related Tools for Reproducible Research in Archaeology
Ben Marwick
Case Study 13: Achieving Full Replication of Our Own Published CFD Results, with Four Diff erent Codes
Olivier Mesnard and Lorena A. Barba
Case Study 14: Reproducible Applied Statistics: Is Tagging of Therapist-Patient Interactions Reliable?
K. Jarrod Millman, Kellie Ottoboni, Naomi A. P. Stark, and Philip B. Stark
Case Study 15: A Dissection of Computational Methods Used in a Biogeographic Study
K. A. S. Mislan
Case Study 16: A Statistical Analysis of Salt and Mortality at the Level of Nations
Kellie Ottoboni
Case Study 17: Reproducible Workflows for Understanding Large-Scale Ecological Effects of Climate Change
Karthik Ram
Case Study 18: Reproducibility in Human Neuroimaging Research: A Practical Example from the Analysis of Diff usion MRI
Ariel Rokem
Case Study 19: Reproducible Computational Science on High-Performance Computers: A View from Neutron Transport
Rachel Slaybaugh
Case Study 20: Detection and Classification of Cervical Cells
Daniela Ushizima
Case Study 21: Enabling Astronomy Image Processing with Cloud Computing Using Apache Spark
Zhao Zhang
PART III: LOW-LEVEL CASE STUDIES
Case Study 22: Software for Analyzing Supernova Light Curve Data for Cosmology
Kyle Barbary
Case Study 23: pyMooney: Generating a Database of Two-Tone Mooney Images
Fatma Deniz
Case Study 24: Problem-Specific Analysis of Molecular Dynamics Trajectories for Biomolecules
Konrad Hinsen
Case Study 25: Developing an Open, Modular Simulation Framework for Nuclear Fuel Cycle Analysis
Kathryn Huff
Case Study 26: Producing a Journal Article on Probabilistic Tsunami Hazard Assessment
Randall J. LeVeque
Case Study 27: A Reproducible Neuroimaging Workflow Using the Automated Build Tool “Make”
Tara Madhyastha, Natalie Koh, and Mary K. Askren
Case Study 28: Generation of Uniform Data Products for AmeriFlux and FLUXNET
Gilberto Pastorello
Case Study 29: Developing a Reproducible Workflow for Large-Scale Phenotyping
Russell Poldrack
Case Study 30: Developing and Testing Stochastic Filtering Methods for Tracking Objects in Videos
Valentina Staneva
Case Study 31: Developing, Testing, and Deploying Efficient MCMC Algorithms for Hierarchical Models Using R
Daniel Turek
Index