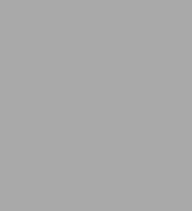
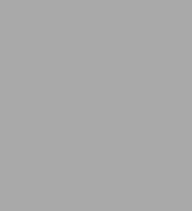
Hardcover(2nd Revised ed.)
-
SHIP THIS ITEMTemporarily Out of Stock Online
-
PICK UP IN STORECheck Availability at Nearby Stores
Available within 2 business hours
Related collections and offers
Overview
Product Details
ISBN-13: | 9781107033252 |
---|---|
Publisher: | Cambridge University Press |
Publication date: | 11/17/2014 |
Series: | Cambridge Handbooks in Psychology |
Edition description: | 2nd Revised ed. |
Pages: | 802 |
Product dimensions: | 6.90(w) x 9.90(h) x 1.60(d) |
About the Author
Read an Excerpt
Cambridge University Press
0521845548 - The Cambridge Handbook of the Learning Sciences - Edited by R. Keith Sawyer
Excerpt
CHAPTER 1
Introduction
The New Science of Learning
R. Keith Sawyer
By the twentieth century, all major industrialized countries offered formal schooling to all of their children. When these schools took shape in the nineteenth and twentieth centuries, scientists didn’t know very much about how people learn. Even by the 1920s, when schools began to become the large bureaucratic institutions that we know today, there still was no sustained study of how people learn. As a result, the schools we have today were designed around commonsense assumptions that had never been tested scientifically:
- Knowledge is a collection of factsabout the world and procedures for how to solve problems. Facts are statements like “The earth is tilted on its axis by 23.45 degrees” and procedures are step-by-step instructions like how to do multidigit addition by carrying to the next column.
- The goal of schooling is to get these facts and procedures into the student’s head. People are considered to be educated when they possess a large collection of these facts and procedures.
- Teachers know these facts and procedures, and their job is to transmit them to students.
- Simpler facts and procedures should be learned first,followed by progressively more complex facts and procedures. The definitions of “simplicity” and “complexity” and the proper sequencing of material were determined either by teachers, by textbook authors, or by asking expert adults like mathematicians, scientists, or historians – not by studying how children actually learn.
- The way to determine the success of schooling is to test students to see how many of these facts and procedures they have acquired.
This traditional vision of schooling is known as instructionism (Papert, 1993). Instructionism prepared students for the industrialized economy of the early twentieth century. But the world today is much more technologically complex and economically competitive, and instructionism is increasingly failing to educate our students to participate in this new kind of society. Economists and organizational theorists have reached a consensus that today we are living in a knowledge economy, an economy that is built on knowledge work (Bereiter, 2002; Drucker, 1993). In the knowledge economy, memorization of facts and procedures is not enough for success. Educated graduates need a deep conceptual understanding of complex concepts, and the ability to work with them creatively to generate new ideas, new theories, new products, and new knowledge. They need to be able to critically evaluate what they read, to be able to express themselves clearly both verbally and in writing, and to be able to understand scientific and mathematical thinking. They need to learn integrated and usable knowledge, rather than the sets of compartmentalized and decontextualized facts emphasized by instructionism. They need to be able to take responsibility for their own continuing, lifelong learning. These abilities are important to the economy, to the continued success of participatory democracy, and to living a fulfilling, meaningful life. Instructionism is particularly ill-suited to the education of creative professionals who can develop new knowledge and continually further their own understanding; instructionism is an anachronism in the modern innovation economy.
Beginning in the 1970s, a new science of learning was born – based in research emerging from psychology, computer science, philosophy, sociology, and other scientific disciplines. As they closely studied children’s learning, scientists discovered that instructionism was deeply flawed. By the 1990s, after about twenty years of research, learning scientists had reached a consensus on the following basic facts about learning – a consensus that was published by the United States National Research Council (see Bransford, Brown, & Cocking, 2000):
- The importance of deeper conceptual understanding. Scientific studies of knowledge workers demonstrate that expert knowledge includes facts and procedures, but simply acquiring those facts and procedures does not prepare a person to perform as a knowledge worker. Factual and procedural knowledge is only useful when a person knows which situations to apply it in, and exactly how to modify it for each new situation. Instructionism results in a kind of learning which is very difficult to use outside of the classroom. When students gain a deeper conceptual understanding, they learn facts and procedures in a much more useful and profound way that transfers to real-world settings.
- Focusing on learning in addition to teaching. Students cannot learn deeper conceptual understanding simply from teachers instructing them better. Students can only learn this by actively participating in their own learning. The new science of learning focuses on student learning processes, as well as instructional technique.
- Creating learning environments. The job of schools is to help students learn the full range of knowledge required for expert adult performance: facts and procedures, of course, but also the deeper conceptual understanding that will allow them to reason about real-world problems. Learning sciences research has identified the key features of those learning environments that help students learn deeper conceptual understanding.
- The importance of building on a learner’s prior knowledge. Learners are not empty vessels waiting to be filled. They come to the classroom with preconceptions about how the world works; some of them are basically correct, and some of them are misconceptions. The best way for children to learn is in an environment that builds on their existing knowledge; if teaching does not engage their prior knowledge, students often learn information just well enough to pass the test, and then revert back to their misconceptions outside of the classroom.
- The importance of reflection. Students learn better when they express their developing knowledge – either through conversation or by creating papers, reports, or other artifacts – and then are provided with opportunities to reflectively analyze their state of knowledge.
This handbook is an introduction to this new science of learning, and how researchers are using that science to lay the groundwork for the schools of the future. This new science is called the learning sciences because it is an interdisciplinary science: it brings together researchers in psychology, education, computer science, and anthropology, among others, and the collaboration among these disciplines has resulted in new ideas, new methodologies, and new ways of thinking about learning. Many people – parents, teachers, policy makers, and even many educational researchers – are not aware of the important discoveries emerging from the learning sciences. Without knowing about the new science of learning, many people continue to assume that schools should be based on instructionism. Parents and policy makers remember being taught that way, and are often uncomfortable when their children have different learning experiences. Many teachers have spent an entire career mastering the skills required to manage an instructionist classroom, and they understandably have trouble envisioning a different kind of school. The purpose of this handbook is to build on the new science of learning by showing various stakeholders how to design learning environments and classrooms:
- For teachers, reading about the new science of learning can help you be more effective in your classrooms.
- For parents, reading about the new science of learning can help you to be an informed consumer of schools. The learning sciences explains why and when instructionism fails and which alternative learning environments are based in contemporary science.
- For administrators, reading about the new science of learning can help you to lead your school into the twenty-first century.
- For policy makers, reading about the new science of learning can help you understand the problems with today’s curricula, teacher education programs, and standardized tests, and how to form a vision for the future.
- For professionals, reading about the new science of learning can help you understand why many people are so poorly informed about science, technology, international relations, economics, and other knowledge-based disciplines.
- And finally, education researchers can learn how their own studies relate to the learning sciences, and can see how to participate in building the schools of the future.
This handbook is the second book that introduces a broad audience to the new science of learning. The first was the NRC report How People Learn, first published in 1999 and with an expanded edition published in 2000 (Bransford, Brown, & Cocking, 2000). That book provides a higher-level overview of the learning sciences; this handbook goes into more depth, is more specific about exactly how to reform schools, and describes important work that has taken place since 1999. In particular, this handbook describes how to use the new sciences of learning to design effective learning environments, in classrooms and outside, often taking advantage of new computer technology. Learning sciences is now over twenty years old; the publication of this handbook is a sign that the scientific community has reached a consensus about some of the most important discoveries about learning. Redesigning schools so that they are based on scientific research is a mammoth undertaking, and it will require the participation of all of the groups that read this book: teachers, parents, school leaders, policy makers, and education researchers.
The Goals of Education and the Nature of Knowledge
The traditional role of educational research has been to tell educators how to achieve their curriculum objectives, but not to help set those objectives. But when learning scientists went into classrooms, they discovered that schools were not teaching the deep knowledge that underlies intelligent performance. By the 1980s, cognitive scientists had discovered that children retain material better, and are able to generalize it to a broader range of contexts, when they learn deep knowledge rather than surface knowledge, and when they learn how to use that knowledge in real-world social and practical settings (see Table 1.1). The notion of deep learning is explored by each learning sciences researcher in a slightly different way, and most of the chapters in this handbook begin by describing the type of deep knowledge studied.
Table 1.1 Deep Learning Versus Traditional Classroom Practices | |
Learning Knowledge Deeply (Findings from Cognitive Science) | Traditional Classroom Practices (Instructionism) |
Deep learning requires that learners relate new ideas and concepts to previous knowledge and experience. | Learners treat course material as unrelated to what they already know. |
Deep learning requires that learners integrate their knowledge into interrelated conceptual systems. | Learners treat course material as disconnected bits of knowledge. |
Deep learning requires that learners look for patterns and underlying principles. | Learners memorize facts and carry out procedures without understanding how or why. |
Deep learning requires that learners evaluate new ideas, and relate them to conclusions. | Learners have difficulty making sense of new ideas that are different from what they encountered in the textbook. |
Deep learning requires that learners understand the process of dialogue through which knowledge is created, and they examine the logic of an argument critically. | Learners treat facts and procedures as static knowledge, handed down from an all-knowing authority. |
Deep learning requires that learners reflect on their own understanding and their own process of learning. | Learners memorize without reflecting on the purpose or on their own learning strategies. |
One of the central underlying themes of the learning sciences is that students learn deeper knowledge when they engage in activities that are similar to the everyday activities of professionals who work in a discipline. Authentic practices are the keystone of many recent educational standards documents in the United States In history, for example, reforms call for learning history by doing historical inquiry rather than memorizing dates and sequences of events: working with primary data sources, and using methods of historical analysis and argumentation that are used by historians (National Center for History in the Schools, 1996). In science, the National Science Education Standards calls for students to engage in the authentic practices of scientific inquiry: constructing explanations and preparing arguments to communicate and justify those explanations (National Research Council, 1996, p. 105).
To better understand how to engage students in authentic practices, many learning sciences reforms are based on studies of professional practice.
- Professionals engage in a process of inquiry, in which they start with a driving question and then use discipline-specific methods to propose hypothetical answers to the question, and to gather and evaluate evidence for and against competing hypotheses (Krajcik & Blumenfeld, this volume; Edelson & Reiser, this volume).
- Professionals use complex representations to communicate with each other during collaboration (as discussed in many of the chapters in Parts 3 and 4).
- Scientists and mathematicians work with concrete, visual models, so students should too (Lehrer & Schauble, this volume).
This focus on authentic practice is based on a new conception of the expert knowledge that underlies knowledge work in today’s economy. In the 1980s and 1990s, scientists began to study science itself, and they began to discover that newcomers become members of a discipline by learning how to participate in all of the practices that are central to professional life in that discipline. And, increasingly, cutting-edge work in the sciences is done at the boundaries of disciplines; for this reason, students need to learn the underlying models, mechanisms, and practices that apply across many scientific disciplines, rather than learning in the disconnected and isolated six-week units that are found in instructionist science classrooms – moving from studying the solar system to studying photosynthesis to studying force and motion, without ever learning about connections among these units.
Studies of knowledge workers show that they almost always apply their expertise in complex social settings, with a wide array of technologically advanced tools along with old-fashioned pencil, paper, chalk, and blackboards. These observations have led learning sciences researchers to a situativity view of knowledge (Greeno, this volume). “Situativity” means that knowledge is not just a static mental structure inside the learner’s head; instead, knowing is a process that involves the person, the tools and other people in the environment, and the activities in which that knowledge is being applied. The situativity perspective moves beyond a transmission and acquisition conception of learning; in addition to acquiring content, what happens during learning is that patterns of participation in collaborative activity change over time (Rogoff, 1990, 1998). This combined research has led the learning sciences to a focus on how children learn in groups (as discussed in the chapters in Part 5).
Of course, students are not capable of doing exactly the same things as highly trained professionals; when learning scientists talk about engaging students in authentic practices, they are referring to developmentally appropriate versions of the situated and meaningful practices of experts. One of the most important goals of learning sciences research is to identify exactly what practices are appropriate for students to engage in and learn, and how learning environments can be designed that are age-appropriate without losing the authenticity of professional practice.
The Foundations of the Learning Sciences
The learning sciences combines many disciplinary approaches to the study of learning. Scholars in a range of university departments conduct research in the learning sciences – they are found in schools of education, of course, but also in departments of computer science and psychology. I review five early influences – constructivism, cognitive science, educational technology, sociocultural studies, and studies of disciplinary knowledge.
Constructivism
In the 1960s and 1970s, Jean Piaget’s writings became widely influential in American education. Before Piaget, most people held to the commonsense belief that children have less knowledge than adults. Piaget argued a radically different theory: although children certainly possess less knowledge than adults, what’s even more important to learning is that children’s minds contain different knowledge structures than are in adults’ minds. In other words, children differ not only in the quantity of knowledge they possess; their knowledge is qualitatively different.
By the 1980s, researchers had confirmed this fundamental claim that children think differently from adults. Educational researchers had discovered, for example, that children don’t get math problems wrong only because they didn’t study hard enough or because they forgot what they read in the textbook – they often got the problems wrong because their minds were thinking about the math problems in a different way than educators expected, and math education wasn’t designed to correct these misconceptions. Cognitive scientists began to identify the cognitive characteristics of children’s “naïve math” and “naïve physics,” and began to accumulate an important body of knowledge about the typical misconceptions that people have about these content areas (diSessa, this volume; Linn, this volume). This body of research allows designers of learning environments to connect learning to students’ prior knowledge and misconceptions.
Constructivism explains why students often do not learn deeply by listening to a teacher, or reading from a textbook. Learning sciences research is revealing the deeper underlying bases of how knowledge construction works. To design effective learning environments, one needs a very good understanding of what children know when they come to the classroom. This requires sophisticated research into children’s cognitive development, and the learning sciences draws heavily on psychological studies of cognitive development (e.g., Siegler, 1998).
Cognitive Science
Many learning scientists began their careers in the interdisciplinary field known as cognitive science. Cognitive science combines experimental investigation of how the mind works (in the tradition of cognitive psychology) with computational modeling of proposed mental processes (in the tradition of artificial intelligence), taking into account what we know from sociology and anthropology about how people use knowledge in everyday settings. Through the 1970s and 1980s, cognitive science did not provide much support to educators, because it focused on laboratory methodologies that removed learners from learning contexts, and because it focused on static knowledge like facts and procedures rather than the processes of thinking and knowing (Kuhn, 1990, p. 1). Around 1990, many key concepts from cognitive science became central in the learning sciences; I discuss representation, expertise, reflection, problem solving, and thinking.
REPRESENTATION
Central to cognitive science is the idea that intelligent behavior is based on representations in the mind: “knowledge structures” such as concepts, beliefs, facts, procedures, and models. In the 1970s, cognitive scientists thought of representation in metaphors drawn from computer memory techniques. A central feature of most computer languages is the pointer: a way for one memory location to “point to” or “refer to” another location. Building on the primitive notion of a pointer, computer programmers were able to develop hierarchically nested data structures – the highest level structure could contain pointers to simpler, lower level structures. For example, the simplest data structure for a house would contain hundreds of variables, including the type of sink in the kitchen and the color of the couch in the living room. But by using the nested data structures that pointers made possible, a more sophisticated data structure for a house could be constructed that would contain pointers to data structures for each room in the house; and the room data structures would each contain pointers to multiple furniture and fixture data structures. This provided a metaphor for how knowledge might be modularized in the mind, and is an example of the kind of metaphors of human cognition that have emerged from computer science.
THE COGNITIVE BASES OF EXPERTISE
One of the most surprising discoveries of 1970s cognitive science was that everyday behavior was harder to represent computationally than expert behavior. Some of the most successful artificial intelligence (AI) programs simulated expert performance in knowledge-intensive domains like medicine, manufacturing, telecommunications, and finance (Liebowitz, 1998). As a result of these efforts, cognitive science developed a sophisticated understanding of the cognitive bases of expertise. Everyday commonsense behavior remains beyond the abilities of AI computer programs, even as some complex aspects of expert performance in knowledge-intensive domains like medicine have been successfully simulated.
A large body of cognitive science research shows that expertise is based on:
- A large and complex set of representational structures
- A large set of procedures and plans
- The ability to improvisationally apply and adapt those plans to each situation’s unique demands
- The ability to reflect on one’s own cognitive processes while they are occurring
REFLECTION
Studies of experts show they are better than novices at planning and criticizing their work – both reflective activities. For example, when expert writers are asked to describe their thought processes out loud as they write, their talk reveals that they develop goals and plans while writing, and they continually reflect on and modify those goals and plans as they write (Flower & Hayes, 1980). School-age writers don’t spend time planning and reflecting (Burtis, Bereiter, Scardamalia, & Tetroe, 1983). Based on these findings, and similar findings regarding other school subjects, learning scientists often conceive of the problem of learning as a problem of transforming novices into experts by developing their ability to reflect on their own thinking in these ways.
Collins and Brown (1988) first suggested that the computer could be used to support reflection (Collins, this volume). Collins and Brown talked about capturing an expert’s process, then allowing the student to compare her process to that of the expert. The computer’s role was to record the expert’s reasoning, making it available whenever it could be useful and to whoever needed it. In this way, the computer was supporting a kind of reflection that was difficult to do without a computer. Since then, several learning sciences projects emphasize computer support for reflection. WISE(Linn, this volume) prompts students to think about evidence and its uses as they are creating a scientific argument. Reciprocal teaching (Palincsar & Brown, 1984) helps students to recognize the questions they need to ask themselves as they are trying to understand something they are reading. Knowledge Forum (Scardamalia & Bereiter, this volume) prompts students to think about their actions and their discussion as they are having knowledge-building conversations. Learning by Design (Kolodner, this volume) integrates reflection into classroom activities.
PROBLEM SOLVING
Cognitive scientists have spent several decades attempting to identify the cognitive bases of problem solving. One of the most persistent theories about problem solving is that it depends on a person having a mental representation of a problem space (Newell & Simon, 1972) which contains beliefs and mental representations – of concepts, specific actions, and the external world. Problem solving is then conceived of as searching through the problem space until the desired goal state is reached. Because knowledge work typically requires problem solving, many learning sciences approaches to learning are based on this research. For example, Koedinger’s cognitive tutors (this volume) assume that production rules are used to move through the problem space, and Kolodner’s case-based reasoning (this volume)assumes that case lookup and matching algorithms are used.
THINKING
Educators often talk about the importance of higher-order thinking skills, but educational programs that emphasize thinking skills are often not based on scientific research. Instead, they are based on one or another intuitively based taxonomy of thinking skills, with almost no scientific justification of why this specific set of skills should be taught in schools (Kuhn, 1990, p. 2). Beginning in the 1980s and 1990s, cognitive psychologists began to study informal reasoning (Voss, Perkins, & Segal, 1991) – the good and bad reasoning that people engage in everyday, when faced with real-life problems that don’t have simple solutions. They also began to study everyday decision making, discovering a wide range of common thinking errors that most people make (Baron, 1985; Kahneman, Slovic, & Tversky, 1982). Also during this time, developmental psychologists began to identify a range of good and bad thinking strategies and how these strategies develop over the lifespan. They extended Piaget’s original insight, showing how children’s thinking differs from that of adults – information that is absolutely critical to education based on the learning sciences (Dunbar & Klahr, 1989; Kuhn, 1989; Schauble, 1990).
Educational Technology
In the 1950s, B. F. Skinner presented his “teaching machines” and claimed that they made the teacher “out of date” (Skinner, 1954/1968, p. 22). The first educational software was designed in the 1960s and was based on Skinner’s behaviorist theories; these systems are known as Computer Assisted Instruction or CAI, and such systems are still in use today. In the 1970s, a few artificial intelligence researchers started working in education, developing automated tutoring systems and other applications (Bobrow & Collins, 1975; Sleeman & Brown, 1982; Wenger, 1987). In the 1980s, cognitive scientists like Roger Schank and Seymour Papert made widely popularized claims that computers would radically transform schools (see Papert, 1980; Schank, this volume).
By the 1990s, a strong consensus had formed among politicians, parents, and the business community that it was essential to get computers into schools (Cuban, 2001). During the 1990s, there was a major push to install computers and the Internet in schools – including federal government programs like E-rate that paid for schools to be connected to the Internet. By 2003, 95 percent of schools were connected to the Internet with high-speed connections, and 93 percent of all classrooms were connected to the Internet. On average, there were 4.4 students for each computer with Internet access; this was a dramatic drop from 12.1 students in 1998, when it was first measured (Parsad & Jones, 2005).
However, the impact of all of this investment has been disappointing. By 2000, no studies had shown that computer use was correlated with improved student performance. When researchers began to look more closely at why computers were having so little impact, they discovered that computer use was not based on the learning sciences; instead, they were being used as quick add-ons to the existing instructional classroom (Cuban, 2001).
Learning scientists emphasize the powerful role that computers can play in transforming all learning. But their vision rejects instructionism and behaviorism and the CAI systems based on it, and presents a new vision of computers in schools. Learning sciences research explains why the promise of computers in schools has not yet been realized; because to date, educational software has been based on instructionist theories, with the computer performing roles that are traditionally performed by the teacher – with the software acting as an expert authority, delivering information to the learner. In contrast, learning sciences suggests that the computer should take on a more facilitating role, helping learners have the kind of experiences that lead to deep learning – for example, helping them to collaborate, or to reflect on their developing knowledge. Many of the chapters in this handbook describe the next generation of educational software, software that is solidly based on the sciences of learning, and that is designed in close collaboration with teachers and schools. Computers are only used as part of overall classroom reform, and only where research shows they will have the most impact. Computer software is central in the learning sciences because the visual and processing power of today’s personal computers supports deep learning:
- Computers can represent abstract knowledge in concrete form
- Computer tools can allow learners to articulate their developing knowledge in a visual and verbal way
- Computers can allow learners to manipulate and revise their developing knowledge via the user interface, in a complex process of design that supports simultaneous articulation, reflection, and learning
- Computers support reflection in a combination of visual and verbal modes
- Internet-based networks of learners can share and combine their developing understandings and benefit from the power of collaborative learning
Sociocultural Studies
After the burst of activity associated with 1970s artificial intelligence and cognitive psychology, by the 1980s many of these scholars had begun to realize that their goal – to understand and simulate human intelligence in the computer – was still very far off. The 1980s disillusionment with AI was so severe that it was informally known as “the AI winter.” Researchers began to step back and think about why the cognitive sciences had not been more successful. The most influential answer was provided by a group of interrelated approaches including the sociocultural, situative, and distributed cognition approaches (Greeno, this volume; Salomon, 1993). Socioculturalists began with the observation that all intelligent behavior was realized in a complex environment – a human created environment filled with tools and machines, but also a deeply social environment with collaborators and partners. Some of the most important studies in this tradition examined how children learn in nonschool settings – how children learn their first language or the norms and conventions of their culture; how apprentices learn on the job. Some of the most interesting work along these lines focused on informal learning in non-Western societies without formal schooling (Cole, 1996; Lave, 1988; Rogoff, 1990; Saxe, 1991). Equally influential studies examined the socially distributed nature of knowledge work – including studies of navy ship navigation (Hutchins, 1995), of London Underground control rooms (Heath & Luff, 1991), of office systems (Suchman, 1987), and of air traffic control centers (Hughes et al., 1988). This research revealed that outside of formal schooling, almost all learning occurs in a complex social environment, and learning is hard to understand if one thinks of it as a mental process occurring within the head of an isolated learner.
The sociocultural approach has been widely influential in all of the disciplines participating in the learning sciences:
- Artificial intelligence began to emphasize “distributed cognition” in part because of the rapidly evolving network technologies of the 1980s and 1990s
- Cognitive psychology began to study teamwork, collaboration, groupdynamics, and the role of social context in cognitive development
- Education research began to study classroom collaboration, collaborative discourse in student groups, and project teams
The Nature of Knowledge Work
Should we reduce auto emissions because of global warming? Should we allow stem cell research to proceed? Should we teach both evolution and creationism in schools? Today’s public debate about such controversial issues shows a glaring lack of knowledge about scientific practice. The U.S. National Science Education Standards (National Research Council, 1996) observed that “Americans are confronted increasingly with questions in their lives that require scientific information and scientific ways of thinking for informed decision making” (p. 11).
By the early 1900s, major industrial countries had all realized the important role that science and engineering played in their rapid growth, and many scholars began to analyze the nature of scientific knowledge. In the first half of the twentieth century, philosophers came to a consensus on the nature of scientific knowledge: scientific knowledge consisted of statements about the world, and logical operations that could be applied to those statements. This consensus was known as logical empiricism (McGuire, 1992; Suppe, 1974). Logical empiricism combined with behaviorism and traditional classroom practice to form the instructionist approach to education: disciplinary knowledge consisted of facts and procedures, and teaching was thought of as transmitting the facts and procedures to students.
Beginning in the 1960s, sociologists, psychologists, and anthropologists began to study how scientists actually did their work, and they increasingly discovered that scientific knowledge was not simply a body of statements and logical operations. In this new view, scientific knowledge is an understanding about how to go about doing science, combined with deep knowledge of models and explanatory principles connected into an integrated conceptual framework. The practice of science involves experimentation, trial and error, hypothesis testing, debate and argumentation. And science is not a solo endeavor; it involves frequent encounters with peers in the scientific community. Scientists frequently talk about evaluating other scientists’ claims, and think about how best to support and present their claims to others.
In this new view, scientific knowledge is situated, practiced, and collaboratively generated. The traditional science classroom, with its lectures and step-by-step lab exercises, completely leaves out these elements of science. But this kind of knowledge would be extremely useful to the general public as they read reports of an experimental drug in the daily paper, as they discuss with their doctor the potential risks of an upcoming surgery, or as they evaluate the health risks of a new development near their neighborhood.
This new view of expert knowledge has been extended beyond science to other forms of knowledge work. For example, literacy scholars have discovered that advanced literacy involves much more than knowing which sounds correspond to which letters; literacy involves knowing how to participate in a complex set of literate practices – like reading a recipe, scanning the classifieds for a specific product, or writing an email to a colleague (Palincsar & Ladewski, this volume). Social science educators have discovered that historians are experts because they know how to engage in the complex practices of historical inquiry and argumentation.
Processes Involved in Learning
The learning sciences are centrally concerned with exactly what is going on in a learning environment, and exactly how it is contributing to improved student performance. The learning environment includes the people in the environment (teachers, learners, and others); the computers in the environment and the roles they play; the architecture and layout of the room and the physical objects in it; and the social and cultural environment. Key questions include: How does learning happen? How do different learning environments contribute to learning, and can we improve the design of learning environments to enhance learning? Some researchers work on specific components of the learning environment – software design, the roles that teachers should play, or specific activities each student performs. Others examine the entire learning environment as a system, and focus on more holistic questions: How much support for the student should come from the teacher, the computer software, or from other students? How can we create a culture where learners feel like a “learning community”? How can we design materials and activities that keep students motivated and sustain their engagement?
© Cambridge University Press