5
1
9780367396220
Subset Selection in Regression / Edition 2 available in Hardcover, Paperback
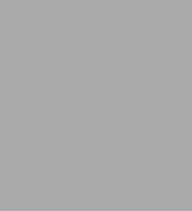
Subset Selection in Regression / Edition 2
- ISBN-10:
- 036739622X
- ISBN-13:
- 9780367396220
- Pub. Date:
- 09/05/2019
- Publisher:
- Taylor & Francis
- ISBN-10:
- 036739622X
- ISBN-13:
- 9780367396220
- Pub. Date:
- 09/05/2019
- Publisher:
- Taylor & Francis
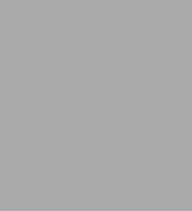
Subset Selection in Regression / Edition 2
$82.99
Current price is , Original price is $82.99. You
82.99
In Stock
Product Details
ISBN-13: | 9780367396220 |
---|---|
Publisher: | Taylor & Francis |
Publication date: | 09/05/2019 |
Series: | Chapman & Hall/CRC Monographs on Statistics and Applied Probability |
Edition description: | 2nd ed. |
Pages: | 256 |
Product dimensions: | 6.00(w) x 9.00(h) x (d) |
About the Author
From the B&N Reads Blog