Statistics And Experimental Design For Psychologists: A Model Comparison Approach
This is the first textbook for psychologists which combines the model comparison method in statistics with a hands-on guide to computer-based analysis and clear explanations of the links between models, hypotheses and experimental designs. Statistics is often seen as a set of cookbook recipes which must be learned by heart. Model comparison, by contrast, provides a mental roadmap that not only gives a deeper level of understanding, but can be used as a general procedure to tackle those problems which can be solved using orthodox statistical methods.Statistics and Experimental Design for Psychologists focusses on the role of Occam's principle, and explains significance testing as a means by which the null and experimental hypotheses are compared using the twin criteria of parsimony and accuracy. This approach is backed up with a strong visual element, including for the first time a clear illustration of what the F-ratio actually does, and why it is so ubiquitous in statistical testing.The book covers the main statistical methods up to multifactorial and repeated measures, ANOVA and the basic experimental designs associated with them. The associated online supplementary material extends this coverage to multiple regression, exploratory factor analysis, power calculations and other more advanced topics, and provides screencasts demonstrating the use of programs on a standard statistical package, SPSS.Of particular value to third year undergraduate as well as graduate students, this book will also have a broad appeal to anyone wanting a deeper understanding of the scientific method.
1125902474
Statistics And Experimental Design For Psychologists: A Model Comparison Approach
This is the first textbook for psychologists which combines the model comparison method in statistics with a hands-on guide to computer-based analysis and clear explanations of the links between models, hypotheses and experimental designs. Statistics is often seen as a set of cookbook recipes which must be learned by heart. Model comparison, by contrast, provides a mental roadmap that not only gives a deeper level of understanding, but can be used as a general procedure to tackle those problems which can be solved using orthodox statistical methods.Statistics and Experimental Design for Psychologists focusses on the role of Occam's principle, and explains significance testing as a means by which the null and experimental hypotheses are compared using the twin criteria of parsimony and accuracy. This approach is backed up with a strong visual element, including for the first time a clear illustration of what the F-ratio actually does, and why it is so ubiquitous in statistical testing.The book covers the main statistical methods up to multifactorial and repeated measures, ANOVA and the basic experimental designs associated with them. The associated online supplementary material extends this coverage to multiple regression, exploratory factor analysis, power calculations and other more advanced topics, and provides screencasts demonstrating the use of programs on a standard statistical package, SPSS.Of particular value to third year undergraduate as well as graduate students, this book will also have a broad appeal to anyone wanting a deeper understanding of the scientific method.
110.0
In Stock
5
1
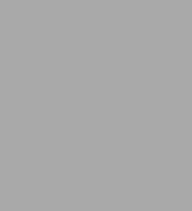
Statistics And Experimental Design For Psychologists: A Model Comparison Approach
472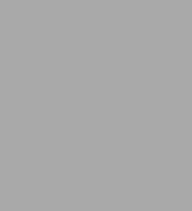
Statistics And Experimental Design For Psychologists: A Model Comparison Approach
472
110.0
In Stock
Product Details
ISBN-13: | 9781786340641 |
---|---|
Publisher: | World Scientific Publishing Europe Ltd |
Publication date: | 10/24/2017 |
Pages: | 472 |
Product dimensions: | 6.00(w) x 9.10(h) x 1.20(d) |
From the B&N Reads Blog