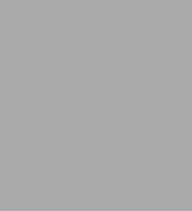
Measurement in Medicine: Philosophical Essays on Assessment and Evaluation
244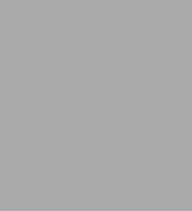
Measurement in Medicine: Philosophical Essays on Assessment and Evaluation
244eBook
Available on Compatible NOOK devices, the free NOOK App and in My Digital Library.
Related collections and offers
Overview
Product Details
ISBN-13: | 9781783488490 |
---|---|
Publisher: | Rowman & Littlefield Publishers, Inc. |
Publication date: | 10/11/2017 |
Sold by: | Barnes & Noble |
Format: | eBook |
Pages: | 244 |
File size: | 1 MB |
Age Range: | 18 Years |
About the Author
Read an Excerpt
CHAPTER 1
How Evidence-Based Medicine Highlights Connections between Measurement and Evidence
Benjamin Smart
Evidence-based medicine (EBM) holds that the evidence provided by epidemiological studies provides better justification for clinical decisions than expert opinion, intuition, or pathological reasoning. It operates on the premise that whether an individual should be treated in a particular way depends primarily upon whether clinical trials have shown the treatment to be beneficial, effective, and cost efficient relative to the alternatives (Sackett et al. 1996). Evidence-based practitioners (those who endorse and practice EBM) thus argue that EBM limits the risk of prescribing inefficient and/or harmful treatments and thus improves mortality and morbidity rates as well as quality of life.
EBM is often associated with a hierarchy of evidence. At the top of the hierarchy sit randomized controlled trials (RCTs), followed by observational studies, such as case-control studies and cohort studies. At the bottom of the evidence hierarchy, one finds mechanistic and pathophysiological reasoning, clinical judgment, and expert opinion/intuition (Cartwright 2007; Howick 2011). Although all respectable forms of medicine are, in some sense, evidence based, the best evidence, as prioritized by the EBM practitioner, is that provided by clinical epidemiologists. Their conclusions are drawn from data collected from carefully designed studies, which, of course, one can obtain only through "measurement"; it is unsurprising, then, that Leah McClimans (2013, 521) has suggested that the paradigm shift to EBM is not so much a shift toward the reliance on evidence as it is a shift toward reliance on measurement.
In this chapter, I highlight how decision making in clinical medicine is vulnerable to implicit bias, but argue that the paradigm shift toward measurement discussed by McClimans goes some way to healing this serious problem in health care. I examine the evidence-based practitioner's approach to evidence in medicine, and in exploring the theory laden nature of both evidence and measurement, I highlight the connection between evidence and measurement that EBM brings into focus.
EVIDENCE-BASED MEDICINE, PATIENT-CENTERED CARE, AND IMPLICIT BIAS
Evidence-Based Medicine
The term "evidence-based medicine" first appeared in a paper by Gordan Guyatt in 1991, but basing clinical decisions on evidence is hardly a new strategy in Western medicine. Indeed, for those skeptical of certain forms of alternative medicine, basing clinical decisions (whether diagnostic, prognostic, or treatment-related) on good evidence is arguably that which distinguishes proper medical practice from that of witch doctors and swindlers.
Crudely speaking, EBM can be viewed as the explicit and judicious use of "best evidence" in a clinical setting (e.g., Sackett 1996). Much, but not all, of this "best" evidence is gathered through measurements in large-scale clinical trials. The evidence obtained translates into best practices and clinical guidelines, which are then implemented by all evidence-based practitioners to try to standardize clinical decisions. This approach is designed to minimize the role of intuition and guesswork in medical practice, which practitioners of EBM assume to be harmful. As I discuss later in this chapter, other forms of evidence are admissible in evidence-based practice, but EBM ultimately dictates that wherever possible, clinical decisions concerning interventions (or lack thereof) should primarily be made in accordance with the clinical guidelines formed in light of RCTs and observational studies, that is, in accordance with the measurements made and interpreted by epidemiologists.
One should note that this is not to say that clinical expertise is irrelevant to evidence-based practice, as some critics of EBM imply (e.g., Karthikeyan and Pais 2010). Good doctors, writes David Sackett and colleagues (1996, 71),
use both individual clinical expertise and the best available external evidence, and neither alone is enough. Without clinical expertise, practice risks becoming tyrannized by evidence, for even excellent external evidence may be inapplicable to or inappropriate for an individual patient. Without current best evidence, practice risks becoming rapidly out of date, to the detriment of patients.
EBM thus requires clinicians to use their clinical expertise to listen to patients, to make the right measurements, and to identify the correct best practices and guidelines to follow.
The alternative paradigm to EBM in modern medicine is "patient-centered care." Clinical decision making, for advocates of patient-centered care, prioritizes the values and needs of the patient and involves more patient participation in treatment decisions. This differs from EBM in that whereas EBM's primary concern is disease (the causes of disease, preventive and curative interventions, and so on), patient-centered care has "a humanistic, biopsychosocial perspective, combining ethical values on the 'ideal physician,' with psychotherapeutic theories on facilitating patients' disclosure of real worries, and negotiation theories on decision making" (Bensing, 2000, 17).
Just as evidence-based practitioners are not entirely robotic in their approach to health care (EBM also respects patients' values; e.g., if a patient is particularly averse to the treatment clinical trials suggest is best [for religious reasons, for example], then evidence-based practitioners will certainly not force that treatment on their patient), those who favor patient-centered care do not altogether ignore biomedical evidence. To ignore all evidence, in this way, would be to rely entirely on luck for both diagnosis and treatment. Nevertheless, EBM's heavier reliance on the evidence produced by clinical trials is indicative of its heavier reliance on measurement.
Implicit Bias and Medicine
Implicit biases, crudely speaking, are unconscious prejudices that affect behavior such that one (unconsciously) discriminates against members of a socially stigmatized group (Brownstein 2015). Although an individual may (and as far as they're concerned, honestly) claim not to be prejudiced against a particular ethnic group, socioeconomic group, or sex, examination of that individual's behavior often indicates otherwise, since social attitudes and stereotypes naturally embed unconscious biases.
Unfortunately, studies have convincingly shown that implicit biases play a significant role in clinical decisions. Green et al. (2007), for example, conducted a study in the United States comparing the thrombolysis decisions for black patients and white patients with acute coronary syndromes. The physicians showed a significant anti-black implicit bias, and further, although their explicit biases did not affect their decisions to treat patients, "as the degree of anti-black bias on the race preference [computer-based implicit association test] increased, recommendations for thrombolysis for black patients decreased" (Green et al. 2007, 1235). Similarly, Schulman et al. (1999) showed that a white female in the United States is far more likely to be recommended for cardiac catheterization by her physician than a black female with the same symptoms. In the United States, at least, the quality of health care received by ethnic minorities thus seems to be significantly lower than that received by their white compatriots (Stepanikova 2012). On the face of it, this can be put down primarily to the implicit biases of clinicians.
In short, despite clinicians' explicit biases tending not to play a role in their clinical decision making, the studies above provide good evidence that clinicians (like the rest of us) are not impervious to implicit bias and, worryingly, these biases are prone to manifest in the form of inconsistent and/or poor clinical decisions.
Implicit Bias and EBM
When a practitioner is inclined toward patient-centered care, her clinical decisions are prima facie particularly vulnerable to implicit bias. Unlike patient-centered care, however, EBM is explicitly designed to encourage (where appropriate) consistency in diagnosis, prognosis, and treatment. On the assumption that the necessary trials have taken place, physicians practicing EBM will (in principle) treat patients with the same symptoms in the same way; that is, assuming (i) that the intervention(s) necessary to treat the condition associated with a set of symptoms has/have been identified by clinical trials and (ii) that these trials have informed the clinical guidelines that evidence-based practitioners are bound to follow, all clinical decisions are standardized so that implicit biases play little or no role in clinical decision making. In principle, then, if all clinicians practiced EBM, situations such as those identified by Schulman et al. (1999) would not arise. On the face of it, at least, the paradigm shift toward measurement is a shift away from the impact of implicit bias in clinical medicine.
IMPLICIT BIAS AND THE THEORY-LADENNESS OF MEASUREMENT IN MEDICINE
Thomas Kuhn argued extensively that scientific observation is theory-laden. What one perceives, deems salient, and describes is at least partly determined by prior scientific theory (Kuhn 1996). To measure velocity of an object, for example, one must at the very least (i) be able to measure (using some device designed for the purpose) the time taken for that object to travel a measured distance; (ii) be able to measure distances accurately; and (iii) understand the theory describing the relationship between time, distance, and velocity. The theory-ladenness of observation, claims Kuhn, extends to all measurements in all sciences.
Good and Bad Theory-Ladenness
Theory-ladenness is often unproblematic. After all, good theories and new technologies permit one to measure outcomes one previously could not. Without magnetic resonance imaging (MRI), for example, much of the information relevant to many patients' cancer treatments would be unobservable (i.e., the development of the theory underlying MRI scanners, and the machine itself, have doubtless saved many lives). The problems concerning theory-ladenness arise when either the theories employed are bad theories or our instruments are not very good at doing what we ask of them. Intuition is a good guide to what counts as good theory and what counts as bad, but nevertheless, below I list some likely qualities of both.
Good Theory-Ladenness
Good theory-ladenness is truth conducive.
Good theory-ladenness enables and/or improves the accuracy of measurement outcomes.
Good theory-ladenness provides higher quality evidence.
Good theory-ladenness is conducive to good clinical decision making.
Bad Theory-Ladenness
Bad theory-ladenness is not truth conducive.
If the theory underlying a measurement is bad, then those measurement outcomes are likely to be inaccurate.
Evidence laden with bad theory is poor evidence.
Bad theory-ladenness is conducive to bad or, at the very least, unjustified clinical decisions.
Given the results of studies such as those considered above, it seems that the stigma attached to groups such as African Americans results in "bad theory." This bad theory manifests in the form of implicit bias, which unconsciously, but systematically, feeds into what clinicians perceive and what is deemed salient to clinical decision making and ultimately can lead to bad decisions concerning prognoses and treatment. As we shall see, EBM attempts to address the consequences of bad forms of theory-ladenness in medicine through additional (strategic) theory.
Kuhn's Three Forms of Theory-Ladenness in Measurement
Consider the following three features of theory-laden observation/measurement identified by Kuhn and their applicability to the medical sciences.
Perceptual Theory Loading
Using the example of combustion, Kuhn notes that when two scientists are working in different paradigms, they perceive different things. The theory of combustion endorsed by Joseph Priestley supposed that combustion and respiration were the release of a substance called phlogiston. Antoine Lavoisier, on the other hand, inspired a paradigm shift to the oxidation theory of combustion. When Priestley observed a substance burning, his perceptual experience conformed to his phlogiston theory; that is, he would (in his mind, at least) perceive the release of phlogiston. But Lavoisier did not share Priestley's perceptual experience. He perceived an oxidation process conforming to his own theory of combustion (e.g., Bogen 2013).
So much for combustion, but how might this apply to medicine? In a discussion concerning the theory-ladenness of perception in psychiatry, Rachel Cooper (2004, 15) discusses studies examining the effect of contextual information on emotion recognition:
[In the studies examined by Fernandez-Dols and Carroll 1997], subjects are shown a face, for example a woman crying, and are given information regarding the context, for example they might be told that the woman has been given a present, and then they are asked to judge the emotion that the person is probably experiencing.
Fernandez-Dols and Carroll's 1997 meta-analysis showed inconsistent results in the trials they studied (i.e., some showed the contextual information to have a significant effect and others did not), so as Cooper points out, when it comes to contextual information of this form we must remain agnostic about the effects of perceptual theory loading. However, both Green et al.'s (2007) study of the effect of pictures depicting the skin color of a participant and Schulman et al.'s (1999) study of treatment differences between black and white women provide significant results. Given that visual stimuli, such as skin color, are present in all clinical consultations, it is reasonable to conclude that perceptual theory loading (minimally in the form of implicit bias) at least sometimes affects clinical decisions.
Semantical Theory Loading
Scientific observations are described using scientific language (e.g., combustion, temperature, mass, velocity, and so on). However, although scientists tend to use the same terms, Kuhn has argued that scientists working within different paradigms understand those terms in different ways. Priestly, when using the word "combustion," understood the term to mean the release of phlogiston. Lavoisier understood it to mean a process of oxidation.
Although I will not defend this claim rigorously, semantical theory loading looks unlikely to be prominent in Western medicine. Medical students in the twenty-first century (in the West) understand scientific terms in roughly the same way since they all operate within a single paradigm. On the other hand, Western medical practitioners, homeopaths, and African traditional healers might understand the scientific terms in different ways, since they operate within different medical paradigms. This chapter is concerned only with modern Western medicine, however, so I will leave this issue alone.
The Aristotelian paradigm, explains Kuhn (1996, 123), requires the experimenter to measure "the weight of the stone, the vertical height to which it had been raised, and the time required for it to reach rest" and "ignore radius, angular displacement, and time per swing." But both angular displacement and time per swing were salient in the Galilean paradigm since that paradigm viewed pendulum swings as constrained circular motions (Bogen 2013). Neither angular displacement nor time per swing is relevant to the Aristotelian paradigm since the Aristotelian views pendula motion as objects "falling under constraint toward the center of the Earth" (Kuhn 1996, 123). Consequently, those working in the Aristotelian paradigm and those working in the Galilean paradigm would measure entirely different quantities when investigating the same phenomenon.
Just as in the above example from physics, practitioners working in different paradigms (e.g., modern medicine, holistic medicine, and traditional healers) are very likely to find different qualities salient and thus make different measurements. Within Western medicine, one might assume there to be a consensus as to what the salient qualities of a patient are, but this is not the case. Those who encourage patient-centered care consider the values, needs, and desires of patients to be far more salient in clinical decision making than do evidence-based practitioners.
(Continues…)
Excerpted from "Measurement in Medicine"
by .
Copyright © 2017 Leah McClimans.
Excerpted by permission of Rowman & Littlefield International, Ltd..
All rights reserved. No part of this excerpt may be reproduced or reprinted without permission in writing from the publisher.
Excerpts are provided by Dial-A-Book Inc. solely for the personal use of visitors to this web site.