Machine Learning: A Constraint-Based Approach provides readers with a refreshing look at the basic models and algorithms of machine learning, with an emphasis on current topics of interest that includes neural networks and kernel machines.
The book presents the information in a truly unified manner that is based on the notion of learning from environmental constraints. While regarding symbolic knowledge bases as a collection of constraints, the book draws a path towards a deep integration with machine learning that relies on the idea of adopting multivalued logic formalisms, like in fuzzy systems. A special attention is reserved to deep learning, which nicely fits the constrained- based approach followed in this book.
This book presents a simpler unified notion of regularization, which is strictly connected with the parsimony principle, and includes many solved exercises that are classified according to the Donald Knuth ranking of difficulty, which essentially consists of a mix of warm-up exercises that lead to deeper research problems. A software simulator is also included.
- Presents fundamental machine learning concepts, such as neural networks and kernel machines in a unified manner
- Provides in-depth coverage of unsupervised and semi-supervised learning
- Includes a software simulator for kernel machines and learning from constraints that also includes exercises to facilitate learning
- Contains 250 solved examples and exercises chosen particularly for their progression of difficulty from simple to complex
Machine Learning: A Constraint-Based Approach provides readers with a refreshing look at the basic models and algorithms of machine learning, with an emphasis on current topics of interest that includes neural networks and kernel machines.
The book presents the information in a truly unified manner that is based on the notion of learning from environmental constraints. While regarding symbolic knowledge bases as a collection of constraints, the book draws a path towards a deep integration with machine learning that relies on the idea of adopting multivalued logic formalisms, like in fuzzy systems. A special attention is reserved to deep learning, which nicely fits the constrained- based approach followed in this book.
This book presents a simpler unified notion of regularization, which is strictly connected with the parsimony principle, and includes many solved exercises that are classified according to the Donald Knuth ranking of difficulty, which essentially consists of a mix of warm-up exercises that lead to deeper research problems. A software simulator is also included.
- Presents fundamental machine learning concepts, such as neural networks and kernel machines in a unified manner
- Provides in-depth coverage of unsupervised and semi-supervised learning
- Includes a software simulator for kernel machines and learning from constraints that also includes exercises to facilitate learning
- Contains 250 solved examples and exercises chosen particularly for their progression of difficulty from simple to complex
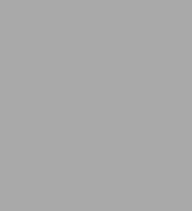
Machine Learning: A Constraint-Based Approach
580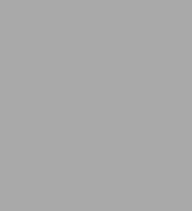
Machine Learning: A Constraint-Based Approach
580Product Details
ISBN-13: | 9780081006702 |
---|---|
Publisher: | Elsevier Science |
Publication date: | 11/20/2017 |
Sold by: | Barnes & Noble |
Format: | eBook |
Pages: | 580 |
File size: | 26 MB |
Note: | This product may take a few minutes to download. |