5
1
9783319663074
Machine Learning for the Quantified Self: On the Art of Learning from Sensory Data available in Hardcover, eBook
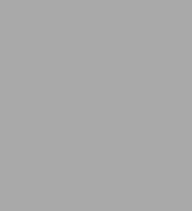
Machine Learning for the Quantified Self: On the Art of Learning from Sensory Data
- ISBN-10:
- 3319663070
- ISBN-13:
- 9783319663074
- Pub. Date:
- 09/29/2017
- Publisher:
- Springer International Publishing
- ISBN-10:
- 3319663070
- ISBN-13:
- 9783319663074
- Pub. Date:
- 09/29/2017
- Publisher:
- Springer International Publishing
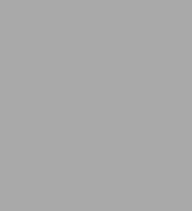
Machine Learning for the Quantified Self: On the Art of Learning from Sensory Data
$199.99
Current price is , Original price is $199.99. You
199.99
In Stock
Product Details
ISBN-13: | 9783319663074 |
---|---|
Publisher: | Springer International Publishing |
Publication date: | 09/29/2017 |
Series: | Cognitive Systems Monographs , #35 |
Edition description: | 1st ed. 2018 |
Pages: | 231 |
Product dimensions: | 6.10(w) x 9.25(h) x (d) |
From the B&N Reads Blog