In a way, the world is made up of approximations, and surely there is no exception in the world of statistics. In fact, approximations, especially large sample approximations, are very important parts of both theoretical and - plied statistics. The Gaussi and is tribution, also known as the normaldistri- tion,is merelyonesuchexample,dueto thewell-knowncentrallimittheorem. Large-sample techniques provide solutions to many practical problems; they simplify our solutions to difficult, sometimes intractable problems; they j- tify our solutions; and they guide us to directions of improvements. On the other hand, just because large-sample approximations are used everywhere, and every day, it does not guarantee that they are used properly, and, when the techniques are misused, there may be serious consequences. 2 Example 1 (Asymptotic distribution). Likelihood ratio test (LRT) is one of the fundamental techniques in statistics. It is well known that, in the 2 “standard” situation, the asymptotic null distribution of the LRT is?,with the degreesoffreedomequaltothe difierencebetweenthedimensions,defined as the numbers of free parameters, of the two nested models being compared (e.g., Rice 1995, pp. 310). This might lead to a wrong impression that the 2 asymptotic (null) distribution of the LRT is always? . A similar mistake 2 might take place when dealing with Pearson’s? -test—the asymptotic distri- 2 2 bution of Pearson’s? -test is not always? (e.g., Moore 1978).
1140409478
Large Sample Techniques for Statistics
In a way, the world is made up of approximations, and surely there is no exception in the world of statistics. In fact, approximations, especially large sample approximations, are very important parts of both theoretical and - plied statistics. The Gaussi and is tribution, also known as the normaldistri- tion,is merelyonesuchexample,dueto thewell-knowncentrallimittheorem. Large-sample techniques provide solutions to many practical problems; they simplify our solutions to difficult, sometimes intractable problems; they j- tify our solutions; and they guide us to directions of improvements. On the other hand, just because large-sample approximations are used everywhere, and every day, it does not guarantee that they are used properly, and, when the techniques are misused, there may be serious consequences. 2 Example 1 (Asymptotic distribution). Likelihood ratio test (LRT) is one of the fundamental techniques in statistics. It is well known that, in the 2 “standard” situation, the asymptotic null distribution of the LRT is?,with the degreesoffreedomequaltothe difierencebetweenthedimensions,defined as the numbers of free parameters, of the two nested models being compared (e.g., Rice 1995, pp. 310). This might lead to a wrong impression that the 2 asymptotic (null) distribution of the LRT is always? . A similar mistake 2 might take place when dealing with Pearson’s? -test—the asymptotic distri- 2 2 bution of Pearson’s? -test is not always? (e.g., Moore 1978).
22.49
In Stock
5
1
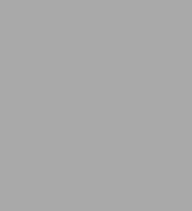
Large Sample Techniques for Statistics
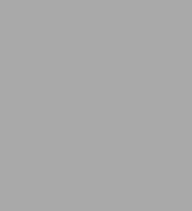
Large Sample Techniques for Statistics
eBook(2nd ed. 2022)
$22.49
$29.99
Save 25%
Current price is $22.49, Original price is $29.99. You Save 25%.
Related collections and offers
22.49
In Stock
Product Details
ISBN-13: | 9783030916954 |
---|---|
Publisher: | Springer-Verlag New York, LLC |
Publication date: | 04/04/2022 |
Series: | Springer Texts in Statistics |
Sold by: | Barnes & Noble |
Format: | eBook |
File size: | 37 MB |
Note: | This product may take a few minutes to download. |
About the Author
From the B&N Reads Blog