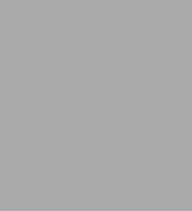
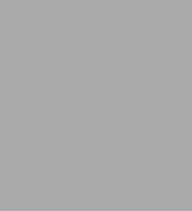
Hardcover
-
PICK UP IN STORECheck Availability at Nearby Stores
Available within 2 business hours
Related collections and offers
Overview
The Internet of Things (IoT) and big data are hot topics in today's interconnected world. They are big contributors to the core problems of information overload and complexity in both military and civilian complex systems and networks. This first-of-its-kind volume reveals the benefits of addressing these topics with the integration of fusion of information and analytics technologies (FIAT). The book explains how FIAT is materialized into decision support systems that are capable of supporting the prognosis, diagnosis, and prescriptive tasks within complex systems and organizations. This unique resource offers keen insight into how complex systems emerge from the interrelation of social and cognitive information, cyber and physical worlds, and the various models of decision-making and situational awareness. Practitioners also discover the central notions of analytics and information fusion.
Product Details
ISBN-13: | 9781630810870 |
---|---|
Publisher: | Artech House, Incorporated |
Publication date: | 01/30/2016 |
Pages: | 312 |
Product dimensions: | 7.20(w) x 10.20(h) x 0.90(d) |
Table of Contents
Preface xiii
Chapter 1 General Context of Cyber Physical and Social Systems 1
1.1 Sociotechnical Organizations and CPSS 1
1.2 CPSS Versus Cyber Physical Systems 1
1.3 CPS Versus Internet of Things (IoT) 4
1.4 Information Overload and the Big Data Problem 5
1.5 Complexity and System Dependability 7
1.6 Fusion of Information and Analytics Technologies (FIAT) 7
1.7 Interface Between the Cyber and Physical Worlds: Four Cybernetic Functions 8
1.8 General Discussion on Decision Support Systems in Complex Dynamic CPSS 9
1.8.1 The Ideal Support System 10
1.8.2 Complex Situations 11
1.8.3 General Overarching System Engineering Concepts in CPSS 11
1.8.4 General Comments About Decision Support for a Smart Grid Environment 13
1.9 Summary 14
References 15
Chapter 2 Situation Awareness and Decision Support 19
2.1 Introduction 19
2.2 Decision-Making in Defense and Security CPSS 20
2.3 Decision-Making Models 24
2.3.1 OODA Loop Interdependencies: Situation Analysis and Decision-Making 27
2.3.2 Selection of the Best Decision-Making Models 29
2.4 Situations and Situation Analysis 30
2.4.1 Complex Events and Information Granules 31
2.4.2 Sense-Making 32
2.5 Situation Awareness 34
2.6 Cognitive System Engineering (CSE) 36
2.6.1 Cognitive Work Analysis (CWA) and Applied CWA 36
2.6.2 Ontological Engineering Versus CWA 38
2.7 Definition of Context 39
2.8 Definitions of Information Fusion and Analytics 40
2.8.1 Joint Directors of Laboratories (JDL) Data Fusion Model 42
2.8.2 Definition of Analytics 44
2.9 Situation Analysis Models (Information Fusion and Analytics) 46
2.9.1 OODA-Based Models 47
2.9.2 The Dual-Node Network (DNN) Architecture of the Revised JDL Model 48
2.9.3 The State Transition Data Fusion (STDF) Model 49
2.9.4 The Interpreted Systems Situation Analysis (ISSA) Approach 50
2.9.5 The Archetypal Dynamics Framework 51
2.10 Awareness Quality and Decision-Making: Information Quality and Quantity 52
2.11 Conclusion 54
References 55
Chapter 3 Information and Uncertainty: Definitions and Representations 63
3.1 Miscellaneous Views on Definition of Information 63
3.2 Semiotics 67
3.3 Information and Uncertainty 68
3.3.1 Information Theory and Entropy 69
3.3.2 Uncertainty and Risk 70
3.3.3 Dequech's Typology of Uncertainty in Economics 73
3.3.4 Dubois and Prade's Typology of Defects 76
3.3.5 Typologies of Uncertainty 77
3.4 Representations of Uncertainty 81
3.4.1 Quantitative Approaches 82
3.4.2 Qualitative Approaches 84
3.4.3 Hybrid and Graphical Approaches 85
3.5 General Uncertainty Principles in Data and Information Transformations 87
3.6 Measures of Uncertainty 88
3.6.1 Requirements for a Measure of Total Uncertainty 91
3.6.2 General Remarks on Measures of Uncertainty 91
3.7 Conclusion 92
References 93
Chapter 4 Information Characterization and Representations 101
4.1 Introduction 101
4.2 Definition of an Information Element 102
4.2.1 Objects, Contents, and Informative Relation 104
4.2.2 Internal/External Contexts 104
4.2.3 Illustrations of (J) at Signal and Data Levels of Abstraction 105
4.2.4 Illustrations of (J) at Higher Levels of Abstraction 107
4.2.5 Basic General Properties of an Information Element 108
4.3 Quality of Information (QoI) 108
4.3.1 The NATO SAS Command and Control (C2) Conceptual Reference Model 110
4.3.2 QoI Framework of Wang and Strong 111
4.3.3 On Defining Ontologies of QoI 112
4.4 Information Element Characterization: Intrinsic Dimensions 112
4.4.1 Information Inner Sets Scope 113
4.4.2 Informative Relation Arity 113
4.4.3 Informative Relation Objectivity 114
4.4.4 Information Imperfections 114
4.5 Contextual Information Characterization 117
4.5.1 Information Reliability 118
4.5.2 Information Consistency 119
4.5.3 Information Relevance 120
4.6 Belief Model Representation 121
4.6.1 Probability Theory 121
4.6.2 Dempster-Shafer Theory 123
4.6.3 Fuzzy Sets Theory 125
4.6.4 Possibility Theory 127
4.6.5 Fuzzy-Evidence Theory (Fuzzy-Belief Structures) 128
4.6.6 Summary on Information Element Representations 130
4.7 Conclusion 131
References 133
Chapter 5 Information Fusion Cells and Processing Strategies 139
5.1 Introduction 139
5.2 Defining an Information Fusion Cell 140
5.3 Processing Strategy 1: Information Fusion Cell, Type 1 (Data Fusion) 142
5.3.1 Building the Fused Information Element 143
5.3.2 Remarks on the Construction of the Joint Informative Relation 145
5.4 Processing Strategy 2: Information Fusion Cell, Type 2 (Parallel Belief Fusion) 146
5.5 Processing Strategy 3: Information Fusion Cell Type 3 (Sequential Belief Fusion) 148
5.5.1 Example 1: Fuzzy Relations Composition 149
5.5.2 Example 2: Belief Transformation 150
5.6 Processing Strategy 4: Information Fusion Cell Type 4 (Competitive Belief Fusion) 152
5.6.1 Dempster Parallel Basic Probability Assignment Combination Rule 153
5.6.2 Hybrid Competitive Belief Models Fusion 154
5.7 Remarks on the Design of Distributed Information Fusion Systems (DIFS) 155
5.8 Conclusion 157
References 157
Chapter 6 A Holonic Processing of Information Fusion Cells 159
6.1 Introduction 159
6.2 Interpretation of Information Elements (as Holons and Informons) 161
6.2.1 Holon Definition Within the Context of Information Fusion 162
6.3 Holon Characterization 164
6.3.1 Types of Holons According to Uncertainty Representations 164
6.4 Information Fusion Cell as a Network of Holons (H) 165
6.4.1 Holonic Information Fusion Core Functions 165
6.5 Quality and Performance of an Information Fusion Cell 169
6.5.1 Desired Information Fusion Cell Properties 170
6.6 Illustration of the Holonic Processing Framework: A Remote-Sensing Case 172
6.6.1 Context and Generation of Informons 172
6.6.2 Illustrative Example: A Remote-Sensing Case 173
6.6.3 JDL Level 1: Objects 173
6.6.4 JDL Level 2: Situations 175
6.6.5 JDL Level 3: Impact 176
6.7 Holonic interpretation of the JDL Model 177
6.7.1 Complex Dynamic Systems 178
6.8 A Holonic Approach for Information Fusion 181
6.8.1 A Holonic View of the JDL Model 181
6.8.2 Examples of Holons and Informons in the JDL's Four Levels 183
6.8.3 Future Work on the Holonic JDL Model 183
6.9 Conclusion 184
References 184
Chapter 7 FIAT-Based Decision Support for Complex Environments 189
7.1 Introduction 189
7.2 System Interoperability in Heterogeneous Environments 191
7.2.1 Barès' Approach to Formalize Cooperability in Military Coalitions 192
7.2.1.4 Barès' Definition of an Interoperability Space 197
7.3 Integrated Frameworks to Process Imperfect Information 204
7.3.1 Integrated Frameworks to Process Imperfect Information 205
7.3.2 Sulis' Causal Tapestries 206
7.3.3 Abstract State Machines (ASM) to Engineer FIAT-Based Systems 206
7.3.3.3 Application Scenario: Tracking of Moving Objects 212
7.3.3.4 The Executable Abstract Model for the Scenario Rendezvous 213
7.4 Conclusion 214
References 215
Chapter 8 FIAT Prospective Research and Future Work 219
8.1 Introduction 219
8.2 Domain Knowledge Representation an d Modeling 219
8.2.1 Ontology Management Methods in Power Network Domain Modeling 220
8.2.2 List of Research Activities for Domain Modeling in Power Networks 221
8.3 Processing of Information and Its Imperfections 222
8.4 Prognosis/Diagnosis/Prescriptive FIAT-Based DSS 224
8.5 Conclusion 227
References 229
Chapter 9 Summary 239
References 241
List of Acronyms 243
About the Authors 247
Index 249