The book discusses the impact of machine learning and computational intelligent algorithms on medical image data processing, and introduces the latest trends in machine learning technologies and computational intelligence for intelligent medical image analysis. The topics covered include automated region of interest detection of magnetic resonance images based on center of gravity; brain tumor detection through low-level features detection; automatic MRI image segmentation for brain tumor detection using the multi-level sigmoid activation function; and computer-aided detection of mammographic lesions using convolutional neural networks.
The book discusses the impact of machine learning and computational intelligent algorithms on medical image data processing, and introduces the latest trends in machine learning technologies and computational intelligence for intelligent medical image analysis. The topics covered include automated region of interest detection of magnetic resonance images based on center of gravity; brain tumor detection through low-level features detection; automatic MRI image segmentation for brain tumor detection using the multi-level sigmoid activation function; and computer-aided detection of mammographic lesions using convolutional neural networks.
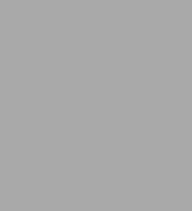
Hybrid Machine Intelligence for Medical Image Analysis
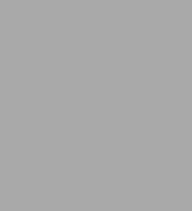
Hybrid Machine Intelligence for Medical Image Analysis
eBook(1st ed. 2020)
Related collections and offers
Product Details
ISBN-13: | 9789811389306 |
---|---|
Publisher: | Springer-Verlag New York, LLC |
Publication date: | 08/08/2019 |
Series: | Studies in Computational Intelligence , #841 |
Sold by: | Barnes & Noble |
Format: | eBook |
File size: | 83 MB |
Note: | This product may take a few minutes to download. |