The 29 contributions presented, as well as an overview paper, were carefully reviewed and selected form numerous submissions. This challenge aims to evaluate and compare the current state-of-the-art methods for automatic head and neck tumor segmentation. In the context of this challenge, a dataset of 325 delineated PET/CT images was made available for training.
The 29 contributions presented, as well as an overview paper, were carefully reviewed and selected form numerous submissions. This challenge aims to evaluate and compare the current state-of-the-art methods for automatic head and neck tumor segmentation. In the context of this challenge, a dataset of 325 delineated PET/CT images was made available for training.
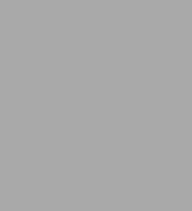
Head and Neck Tumor Segmentation and Outcome Prediction: Second Challenge, HECKTOR 2021, Held in Conjunction with MICCAI 2021, Strasbourg, France, September 27, 2021, Proceedings
328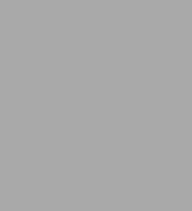
Head and Neck Tumor Segmentation and Outcome Prediction: Second Challenge, HECKTOR 2021, Held in Conjunction with MICCAI 2021, Strasbourg, France, September 27, 2021, Proceedings
328Paperback(1st ed. 2022)
Product Details
ISBN-13: | 9783030982522 |
---|---|
Publisher: | Springer International Publishing |
Publication date: | 03/13/2022 |
Series: | Lecture Notes in Computer Science , #13209 |
Edition description: | 1st ed. 2022 |
Pages: | 328 |
Product dimensions: | 6.10(w) x 9.25(h) x (d) |