5
1
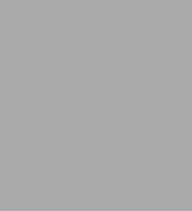
Hands-On Graph Neural Networks Using Python: Practical techniques and architectures for building powerful graph and deep learning apps with PyTorch
354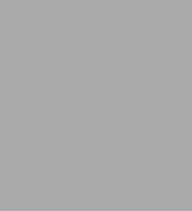
Hands-On Graph Neural Networks Using Python: Practical techniques and architectures for building powerful graph and deep learning apps with PyTorch
354eBook
$29.99
$39.99
Save 25%
Current price is $29.99, Original price is $39.99. You Save 25%.
Related collections and offers
29.99
In Stock
Product Details
ISBN-13: | 9781804610701 |
---|---|
Publisher: | Packt Publishing |
Publication date: | 04/14/2023 |
Sold by: | Barnes & Noble |
Format: | eBook |
Pages: | 354 |
File size: | 16 MB |
Note: | This product may take a few minutes to download. |
About the Author
From the B&N Reads Blog