5
1
9781493951666
Handbook of Simulation Optimization available in Hardcover, Paperback, eBook
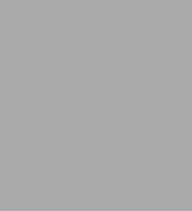
Handbook of Simulation Optimization
- ISBN-10:
- 1493951661
- ISBN-13:
- 9781493951666
- Pub. Date:
- 08/23/2016
- Publisher:
- Springer New York
- ISBN-10:
- 1493951661
- ISBN-13:
- 9781493951666
- Pub. Date:
- 08/23/2016
- Publisher:
- Springer New York
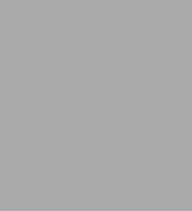
Handbook of Simulation Optimization
$179.99
Current price is , Original price is $179.99. You
179.99
In Stock
Product Details
ISBN-13: | 9781493951666 |
---|---|
Publisher: | Springer New York |
Publication date: | 08/23/2016 |
Series: | International Series in Operations Research & Management Science , #216 |
Edition description: | Softcover reprint of the original 1st ed. 2015 |
Pages: | 387 |
Product dimensions: | 6.10(w) x 9.25(h) x 0.03(d) |
About the Author
From the B&N Reads Blog