“Simon Hubbert has written an excellent introduction to the mathematical foundations of market risk management. His book is written in an elegant style, striking the balance between complexity and accessibility. Great attention has been given to providing a clear exposition of the scientific concepts behind the subject. It should be top of the list for anyone seeking a thorough account of how mathematics can be used to solve complex problems in financial risk management.”
—Helyette Geman, Professor of Finance and Director of the Commodity Finance Centre, Birkbeck, University of London & ESCP Europe
“In a manner reminiscent of his lectures Dr Hubbert not only makes detailed reference to the mathematical and statistical premises underlying the methodology of financial risk management but unlike most books in this arena goes on to explain and expand on those premises in a clear and detailed fashion. This book addresses a key gap in the current literature and will provide an important reference for both students and practitioners alike.”
—Philip Brown, MSc Financial Engineering, BSc (Hons) Mathematics, CVA Analyst
“This well-organised book succeeds in expressing clearly many fundamental ideas that are often expressed very cloudily in standard finance texts. It is both accessible and rigorous at the same time.”
—Steve Satchell, Fellow, Trinity College, University of Cambridge
“This book is a very comprehensive and well structured resource, providing all the mathematics behind market risk management. Throughout the book the reader benefits from Simon’s experiences across academia and the banking industry. It is mathematically sound, but not just a theoretical handbook – it also covers the practical risk management requirements as they appear in today’s markets, and discusses the limitations of models as well. This is an excellent book that will accompany me as a reference on mathematical concepts for market risk management.”
—Dr Bernhard Napiontek, Head of Risk Management Consulting, IBM Global Business Services, Germany
“Simon Hubbert has written an excellent introduction to the mathematical foundations of market risk management. His book is written in an elegant style, striking the balance between complexity and accessibility. Great attention has been given to providing a clear exposition of the scientific concepts behind the subject. It should be top of the list for anyone seeking a thorough account of how mathematics can be used to solve complex problems in financial risk management.”
—Helyette Geman, Professor of Finance and Director of the Commodity Finance Centre, Birkbeck, University of London & ESCP Europe
“In a manner reminiscent of his lectures Dr Hubbert not only makes detailed reference to the mathematical and statistical premises underlying the methodology of financial risk management but unlike most books in this arena goes on to explain and expand on those premises in a clear and detailed fashion. This book addresses a key gap in the current literature and will provide an important reference for both students and practitioners alike.”
—Philip Brown, MSc Financial Engineering, BSc (Hons) Mathematics, CVA Analyst
“This well-organised book succeeds in expressing clearly many fundamental ideas that are often expressed very cloudily in standard finance texts. It is both accessible and rigorous at the same time.”
—Steve Satchell, Fellow, Trinity College, University of Cambridge
“This book is a very comprehensive and well structured resource, providing all the mathematics behind market risk management. Throughout the book the reader benefits from Simon’s experiences across academia and the banking industry. It is mathematically sound, but not just a theoretical handbook – it also covers the practical risk management requirements as they appear in today’s markets, and discusses the limitations of models as well. This is an excellent book that will accompany me as a reference on mathematical concepts for market risk management.”
—Dr Bernhard Napiontek, Head of Risk Management Consulting, IBM Global Business Services, Germany
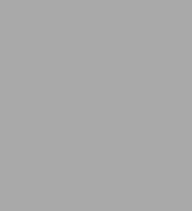
Essential Mathematics for Market Risk Management
352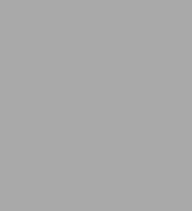
Essential Mathematics for Market Risk Management
352Related collections and offers
Product Details
ISBN-13: | 9781119953029 |
---|---|
Publisher: | Wiley |
Publication date: | 12/12/2011 |
Series: | The Wiley Finance Series , #642 |
Sold by: | JOHN WILEY & SONS |
Format: | eBook |
Pages: | 352 |
File size: | 11 MB |
Note: | This product may take a few minutes to download. |