Simplify data science infrastructure to give data scientists an efficient path from prototype to production.
In Effective Data Science Infrastructure you will learn how to:
Design data science infrastructure that boosts productivity
Handle compute and orchestration in the cloud
Deploy machine learning to production
Monitor and manage performance and results
Combine cloud-based tools into a cohesive data science environment
Develop reproducible data science projects using Metaflow, Conda, and Docker
Architect complex applications for multiple teams and large datasets
Customize and grow data science infrastructure
Effective Data Science Infrastructure: How to make data scientists more productive is a hands-on guide to assembling infrastructure for data science and machine learning applications. It reveals the processes used at Netflix and other data-driven companies to manage their cutting edge data infrastructure. In it, you’ll master scalable techniques for data storage, computation, experiment tracking, and orchestration that are relevant to companies of all shapes and sizes. You’ll learn how you can make data scientists more productive with your existing cloud infrastructure, a stack of open source software, and idiomatic Python.
The author is donating proceeds from this book to charities that support women and underrepresented groups in data science.
About the technology
Growing data science projects from prototype to production requires reliable infrastructure. Using the powerful new techniques and tooling in this book, you can stand up an infrastructure stack that will scale with any organization, from startups to the largest enterprises.
About the book
Effective Data Science Infrastructure teaches you to build data pipelines and project workflows that will supercharge data scientists and their projects. Based on state-of-the-art tools and concepts that power data operations of Netflix, this book introduces a customizable cloud-based approach to model development and MLOps that you can easily adapt to your company’s specific needs. As you roll out these practical processes, your teams will produce better and faster results when applying data science and machine learning to a wide array of business problems.
What's inside
Handle compute and orchestration in the cloud
Combine cloud-based tools into a cohesive data science environment
Develop reproducible data science projects using Metaflow, AWS, and the Python data ecosystem
Architect complex applications that require large datasets and models, and a team of data scientists
About the reader
For infrastructure engineers and engineering-minded data scientists who are familiar with Python.
About the author
At Netflix, Ville Tuulos designed and built Metaflow, a full-stack framework for data science. Currently, he is the CEO of a startup focusing on data science infrastructure.
Table of Contents
1 Introducing data science infrastructure
2 The toolchain of data science
3 Introducing Metaflow
4 Scaling with the compute layer
5 Practicing scalability and performance
6 Going to production
7 Processing data
8 Using and operating models
9 Machine learning with the full stack
"1140636464"
In Effective Data Science Infrastructure you will learn how to:
Design data science infrastructure that boosts productivity
Handle compute and orchestration in the cloud
Deploy machine learning to production
Monitor and manage performance and results
Combine cloud-based tools into a cohesive data science environment
Develop reproducible data science projects using Metaflow, Conda, and Docker
Architect complex applications for multiple teams and large datasets
Customize and grow data science infrastructure
Effective Data Science Infrastructure: How to make data scientists more productive is a hands-on guide to assembling infrastructure for data science and machine learning applications. It reveals the processes used at Netflix and other data-driven companies to manage their cutting edge data infrastructure. In it, you’ll master scalable techniques for data storage, computation, experiment tracking, and orchestration that are relevant to companies of all shapes and sizes. You’ll learn how you can make data scientists more productive with your existing cloud infrastructure, a stack of open source software, and idiomatic Python.
The author is donating proceeds from this book to charities that support women and underrepresented groups in data science.
About the technology
Growing data science projects from prototype to production requires reliable infrastructure. Using the powerful new techniques and tooling in this book, you can stand up an infrastructure stack that will scale with any organization, from startups to the largest enterprises.
About the book
Effective Data Science Infrastructure teaches you to build data pipelines and project workflows that will supercharge data scientists and their projects. Based on state-of-the-art tools and concepts that power data operations of Netflix, this book introduces a customizable cloud-based approach to model development and MLOps that you can easily adapt to your company’s specific needs. As you roll out these practical processes, your teams will produce better and faster results when applying data science and machine learning to a wide array of business problems.
What's inside
Handle compute and orchestration in the cloud
Combine cloud-based tools into a cohesive data science environment
Develop reproducible data science projects using Metaflow, AWS, and the Python data ecosystem
Architect complex applications that require large datasets and models, and a team of data scientists
About the reader
For infrastructure engineers and engineering-minded data scientists who are familiar with Python.
About the author
At Netflix, Ville Tuulos designed and built Metaflow, a full-stack framework for data science. Currently, he is the CEO of a startup focusing on data science infrastructure.
Table of Contents
1 Introducing data science infrastructure
2 The toolchain of data science
3 Introducing Metaflow
4 Scaling with the compute layer
5 Practicing scalability and performance
6 Going to production
7 Processing data
8 Using and operating models
9 Machine learning with the full stack
Effective Data Science Infrastructure: How to make data scientists productive
Simplify data science infrastructure to give data scientists an efficient path from prototype to production.
In Effective Data Science Infrastructure you will learn how to:
Design data science infrastructure that boosts productivity
Handle compute and orchestration in the cloud
Deploy machine learning to production
Monitor and manage performance and results
Combine cloud-based tools into a cohesive data science environment
Develop reproducible data science projects using Metaflow, Conda, and Docker
Architect complex applications for multiple teams and large datasets
Customize and grow data science infrastructure
Effective Data Science Infrastructure: How to make data scientists more productive is a hands-on guide to assembling infrastructure for data science and machine learning applications. It reveals the processes used at Netflix and other data-driven companies to manage their cutting edge data infrastructure. In it, you’ll master scalable techniques for data storage, computation, experiment tracking, and orchestration that are relevant to companies of all shapes and sizes. You’ll learn how you can make data scientists more productive with your existing cloud infrastructure, a stack of open source software, and idiomatic Python.
The author is donating proceeds from this book to charities that support women and underrepresented groups in data science.
About the technology
Growing data science projects from prototype to production requires reliable infrastructure. Using the powerful new techniques and tooling in this book, you can stand up an infrastructure stack that will scale with any organization, from startups to the largest enterprises.
About the book
Effective Data Science Infrastructure teaches you to build data pipelines and project workflows that will supercharge data scientists and their projects. Based on state-of-the-art tools and concepts that power data operations of Netflix, this book introduces a customizable cloud-based approach to model development and MLOps that you can easily adapt to your company’s specific needs. As you roll out these practical processes, your teams will produce better and faster results when applying data science and machine learning to a wide array of business problems.
What's inside
Handle compute and orchestration in the cloud
Combine cloud-based tools into a cohesive data science environment
Develop reproducible data science projects using Metaflow, AWS, and the Python data ecosystem
Architect complex applications that require large datasets and models, and a team of data scientists
About the reader
For infrastructure engineers and engineering-minded data scientists who are familiar with Python.
About the author
At Netflix, Ville Tuulos designed and built Metaflow, a full-stack framework for data science. Currently, he is the CEO of a startup focusing on data science infrastructure.
Table of Contents
1 Introducing data science infrastructure
2 The toolchain of data science
3 Introducing Metaflow
4 Scaling with the compute layer
5 Practicing scalability and performance
6 Going to production
7 Processing data
8 Using and operating models
9 Machine learning with the full stack
In Effective Data Science Infrastructure you will learn how to:
Design data science infrastructure that boosts productivity
Handle compute and orchestration in the cloud
Deploy machine learning to production
Monitor and manage performance and results
Combine cloud-based tools into a cohesive data science environment
Develop reproducible data science projects using Metaflow, Conda, and Docker
Architect complex applications for multiple teams and large datasets
Customize and grow data science infrastructure
Effective Data Science Infrastructure: How to make data scientists more productive is a hands-on guide to assembling infrastructure for data science and machine learning applications. It reveals the processes used at Netflix and other data-driven companies to manage their cutting edge data infrastructure. In it, you’ll master scalable techniques for data storage, computation, experiment tracking, and orchestration that are relevant to companies of all shapes and sizes. You’ll learn how you can make data scientists more productive with your existing cloud infrastructure, a stack of open source software, and idiomatic Python.
The author is donating proceeds from this book to charities that support women and underrepresented groups in data science.
About the technology
Growing data science projects from prototype to production requires reliable infrastructure. Using the powerful new techniques and tooling in this book, you can stand up an infrastructure stack that will scale with any organization, from startups to the largest enterprises.
About the book
Effective Data Science Infrastructure teaches you to build data pipelines and project workflows that will supercharge data scientists and their projects. Based on state-of-the-art tools and concepts that power data operations of Netflix, this book introduces a customizable cloud-based approach to model development and MLOps that you can easily adapt to your company’s specific needs. As you roll out these practical processes, your teams will produce better and faster results when applying data science and machine learning to a wide array of business problems.
What's inside
Handle compute and orchestration in the cloud
Combine cloud-based tools into a cohesive data science environment
Develop reproducible data science projects using Metaflow, AWS, and the Python data ecosystem
Architect complex applications that require large datasets and models, and a team of data scientists
About the reader
For infrastructure engineers and engineering-minded data scientists who are familiar with Python.
About the author
At Netflix, Ville Tuulos designed and built Metaflow, a full-stack framework for data science. Currently, he is the CEO of a startup focusing on data science infrastructure.
Table of Contents
1 Introducing data science infrastructure
2 The toolchain of data science
3 Introducing Metaflow
4 Scaling with the compute layer
5 Practicing scalability and performance
6 Going to production
7 Processing data
8 Using and operating models
9 Machine learning with the full stack
43.99
In Stock
5
1
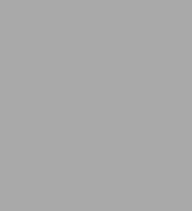
Effective Data Science Infrastructure: How to make data scientists productive
352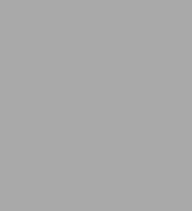
Effective Data Science Infrastructure: How to make data scientists productive
352Related collections and offers
43.99
In Stock
From the B&N Reads Blog