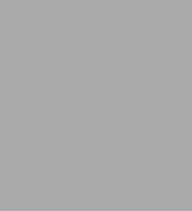
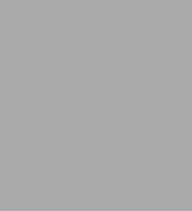
eBook
Available on Compatible NOOK devices, the free NOOK App and in My Digital Library.
Related collections and offers
Overview
Leonelli is the first scholar to use a study of contemporary data-intensive science to provide a philosophical analysis of the epistemology of data. In analyzing the rise, internal dynamics, and potential impact of data-centric biology, she draws on scholarship across diverse fields of science and the humanities—as well as her own original empirical material—to pinpoint the conditions under which digitally available data can further our understanding of life. Bridging the divide between historians, sociologists, and philosophers of science, Data-Centric Biology offers a nuanced account of an issue that is of fundamental importance to our understanding of contemporary scientific practices.
Product Details
ISBN-13: | 9780226416502 |
---|---|
Publisher: | University of Chicago Press |
Publication date: | 11/18/2016 |
Sold by: | Barnes & Noble |
Format: | eBook |
Pages: | 288 |
File size: | 3 MB |
About the Author
Read an Excerpt
Data-Centric Biology
A Philosophical Study
By Sabina Leonelli
The University of Chicago Press
Copyright © 2016 The University of ChicagoAll rights reserved.
ISBN: 978-0-226-41650-2
CHAPTER 1
Making Data Travel: Technology and Expertise
On the morning of September 17, 2013, I made my way to the University of Warwick to attend a workshop called "Data Mining with iPlant." The purpose of the workshop was to teach UK plant biologists how to use the iPlant Collaborative, a digital platform funded by the National Science Foundation in the United States to provide digital tools for the storage, analysis, and interpretation of plant science data. iPlant is a good example of the kind of technology, and related research practices, whose epistemic significance this book aims to explore. It is a digital infrastructure developed in order to make various types of biological data travel far and wide, so that those data can be analyzed by several groups of scientists across the globe, integrated with yet more data, and ultimately help biologists to generate new knowledge. While recognizing that gathering all existing plant data under one roof is a hopelessly ambitious goal, iPlant aims to incorporate as many data types — ranging from genetic to morphological and ecological — about as many plant species as possible. It also aims to develop software that plant biologists can easily learn to use for their own research purposes, thus minimizing the amount of specialized training needed to access the resource and facilitating its interactions with other digital services and databases.
The iPlant staff, which comprises over fifty individuals with expertise in both computer science and experimental biology, took a few years to get started on this daunting project. This is because setting up a digital infrastructure to support scientific inquiry involves tackling substantial challenges involving the collection, handling, and dissemination of data across a wide variety of fields, as well as devising appropriate software to handle user demands. Initially, iPlant staff had to determine which features of data analysis are most valued and urgently needed by plant scientists, so as to establish which goals to tackle and in which order. At the outset of the project in 2008, a substantial portion of funding was therefore devoted to consultations with members of the plant science community worldwide in order to ascertain their requirements and preferences. iPlant staff then focused on making these ideas practically and computationally feasible given the technology, manpower, and data collections at hand. They organized the physical spaces and equipment needed to store and manage very large files, including adequate computing facilities, servers powerful enough to support the operations at hand, and work stations for the dozens of staff and technicians involved across several campuses in Texas, California, Arizona, and New York. They also developed software for the management and analysis of data, which would support teams based at different locations and the integration of data of various formats and provenance. These efforts led to even more consultations with biologists (to check whether the solutions singled out by iPlant would be acceptable) as well as many groups involved in building the resource, such as the software developers, storage service providers, mathematicians, and programmers. The first version of the iPlant user interface, called Discovery Environment, was not released until 2011.
Plant scientists around the world watched these developments with anticipation in the hope of learning new ways to search existing data sets and make sense of their own data. The workshop at Warwick was thus well attended, as most plant science groups in the United Kingdom sent representatives to the meeting. It was held in a brand-new computer room situated at the center of the life sciences building — a typical instance of the increasing prominence of biological research performed through computer analysis over "wet" experiments on organic materials. I took my place at one of the 120 large iMacs populating the room and set out to perform introductory exercises devised by iPlant staff to get biologists acquainted with their tools. After the first hour, I started to perceive some restless shuffling near me. Some of the biologists were getting impatient with the amount of coding and programming involved in the exercises and protesting to their neighbors that the data analysis they were hoping to carry out did not seem to be feasible within that system. Indeed, far from being able to use iPlant to their research advantage, they were getting stuck with tasks such as uploading their own data into the iPlant system, understanding which data formats worked with the visualization tools available in the Discovery Environment, and customizing parameters to fit existing research goals — and becoming frustrated as a result.
This impatience may appear surprising. These biologists were attending this workshop precisely to acquaint themselves with the programs used to power the computational tools offered by iPlant in anticipation of eventually contributing to their development — indeed, the labs had selected their most computationally oriented staff members as delegates for this event. Furthermore, as iPlant coordinators kept repeating throughout the day, those tools were the result of ongoing efforts to make the interface used for data analysis flexible to new uses and accessible to researchers with limited computer skills, and iPlant staff was at hand to help with specific queries and problems (in the words of iPlant co–principal investigator Dan Stanzione, "We are here to enable users to do their thing"). Yet I could understand the unease felt by biologists struggling with the limits and challenges of iPlant tools and the learning curve required to use them to their full potential. Like them, I had read the "manifesto" paper in which iPlant developers explained their activities, and I had been struck by the simplicity and power of their vision. The paper starts with an imaginary user scenario: Tara, a biologist interested in environmental susceptibility of plant genomes, uses iPlant software to seamlessly integrate data across various formats, thousands of genomes, and hundreds of species, which ultimately enables her to identify new patterns and causal relations between key biological components and processes. This example vividly illustrates how data infrastructure could help understand how processes at the molecular level affect, and are in turn affected by, the behavior, morphology, and environment of organisms. Advances in this area have the potential to foster scientific solutions to what Western governments call the "grand challenges" of our time, such as the need to feed the rapidly increasing world population by growing plants more efficiently. As is often the case with large data infrastructures set up in the early 2000s, the stakes involved in the expectations set up by iPlant are as high as they can be. It is understandable that after reading that manifesto paper, biologists attending the workshop got frustrated when confronted with the challenges involved in getting iPlant to work and the limitations in the types of analyses that iPlant could handle.
This tension between promise and reality, between what data technologies can achieve in principle and what it takes to make them work in practice, is inescapable when analyzing any instance of data-centric research and constitutes the starting point for my study. On the one hand, iPlant exemplifies what many biologists see as a brave new research world, in which the billions of data churned out by high-throughput machines can be integrated with experimentally generated data, leading to an overall understanding of how organisms function and relate to each other. On the other hand, developing digital databases that can support this vision requires the coordination of diverse skills, interests, and backgrounds to match the wide variety of data types, research scenarios, and expertises involved. Such coordination is achieved through what I will call packaging procedures for data, which include data selection, formatting, standardization, and classification, as well as the development of methods for retrieval, analysis, visualization, and quality control. These procedures constitute the backbone of data-centric research. Inadequate packaging makes it impossible to integrate and mine data, thus calling into question the plausibility of the promises made by the developers of large data infrastructures. As exemplified by the lengthy negotiations surrounding the development of iPlant, efforts to develop adequate packaging involve critical reflection over the conditions under which data dissemination, integration, and interpretation can or should take place and who should be involved in making them possible.
In this chapter, I examine the unresolved tensions, practical challenges, and creative solutions involved in packaging data for dissemination. I focus on the procedures involved in labeling data for retrieval within model organism databases. These databases constitute an exceptionally sophisticated attempt to support data integration and reuse, which is rooted in the history of twentieth-century life science, particularly the rise of molecular biology in the 1960s and large-scale sequencing projects in the 1990s. In contrast to infrastructures such as GenBank that only cater for one data type, they are meant to store a variety of automatically produced and experimentally obtained data and make them accessible to research groups with markedly different epistemic cultures. I explore the wealth and diversity of resources that these databases draw on to fulfill their complex mandate and identify two processes without which data could not travel outside of their original context of production: decontextualization and recontextualization. I then discuss how the introduction of computational tools to disseminate large data sets is reconfiguring the skills and expertise associated with biological research through the emergence of a new professional figure: the database curator. Finally, I introduce the notion of data journeys and reflect on the significance of using metaphors relating to movement and travel when examining data dissemination practices.
1.1 The Rise of Online Databases in Biology
In the period following the Second World War, biology entered a molecular bandwagon. Starting from the 1960s, biochemistry and genetics absorbed the vast majority of investments and public attention allocated to biology, culminating in the genome sequencing projects of the 1990s. These projects, which included the Human Genome Project and initiatives centered on nonhuman organisms such as the bacterium Escherichia coli and the plant Arabidopsis thaliana, were ostensibly aimed at "deciphering the code of life" by finding ways to map and document the string of nucleotides contained in the genome. Many biologists cast doubt over their overall usefulness and scientific significance, and philosophers condemned the commitment to genetic reductionism that these projects seemed to support (namely, the idea that life can be understood primarily by reference to molecular processes by determining the order of nucleotides within a DNA molecule). Despite these objections, these projects were very well funded and the rhetoric of coding and mapping life captured the imagination of national governments and media outlets, resulting in a public visibility rarely enjoyed by scientific initiatives.
When many sequencing projects announced completion in the early 2000s, it became clear that they had indeed yielded little biological insight into the functioning of organisms, particularly when compared to the hype and expectations surrounding their initial funding. This fact in itself can be viewed as a significant outcome. It demonstrated that sequence data are an important achievement but do not suffice to yield an improved understanding of life. Rather, they need to be integrated with data documenting other biological components, processes, and levels of organization, such as, for instance, data acquired within cell and developmental biology, physiology, and ecology. Sequencing projects can thus be seen as spelling the end of genetic reductionism in biology and opening the door to holistic, integrative approaches to organisms as "complex wholes" such as those touted within systems biology.
Another major outcome of sequencing projects was their success in bringing the scientific importance of activities of data production, dissemination, and integration to the attention of biologists, funding agencies, and governments. Despite their central role throughout the history of biological research, practices of data collection and dissemination have not enjoyed a high status and visibility outside narrow circles of experts. Developing a smart archive or a way to store samples would typically be regarded as a technical contribution, rather than as a contribution to scientific knowledge, and only when those tools were used to generate new claims about the world would the word "science" be invoked. As I stressed in the introduction, this situation has changed over the last decades, and it is no coincidence that such a shift in the prominence of data practices has coincided with the elevation of sequencing from mere technology to a scientific specialty in its own right, requiring targeted expertise and skills.
As highlighted by Hallam Stevens in his book Life Out of Sequence, the data produced by sequencing projects have come to exemplify the idea of "big data" in biology. It is in the context of sequencing projects that high-throughput machines, able to generate large quantities of data points with minimal human intervention, were developed. These projects produced vast data sets documenting the genotypes of organisms, which in turn fueled debate on how these data could be stored, whether they could be efficiently shared, and how they could be integrated with data that were not generated in a similarly automated manner. They generated interest in producing data about other aspects of subcellular biology, most prominently through "omics" data including metabolomics (documenting metabolite behavior), transcriptomics (gene expression), and proteomics (protein structure and functions). Furthermore, sequencing projects established a template for how the international research community could cooperate, particularly in the form of large-scale projects and networks through which access, use, and maintenance of machines and data infrastructures was structured and regimented. Finally, sequence data became a classic example of biological data produced in the absence of a specific research question, as ways of exploring the molecular features of a given organism rather than testing a given hypothesis. More than their sheer size and speed of production, this disconnection between research questions and data generation is what marks sequences out as big data. As in comparable cases in particle physics, meteorology, and astronomy, the acquisition of these data required large efforts and diverted investments from other areas of inquiry, and yet there was no certainty as to whether and how sequence data could be used to deliver the promised biological and medical breakthroughs — a situation that generated high levels of anxiety among funders and members of the scientific public, as well as a sense of urgency about finding ways to analyze and interpret the data.
This space of opportunity mixed with anxiety proved decisive to the development and scientific success of model organism databases. To explain how this came about, I need to briefly introduce the key characteristics of model organisms and reflect on their history as laboratory materials. Model organisms are a small number of species, including the fruit fly (Drosophila melanogaster), the nematode (Caenorhabditis elegans), the zebrafish (Danio rerio), the budding yeast (Saccharomyces cerevisiae), the weed (Arabidopsis thaliana), and the house mouse (Mus musculus), whose study has absorbed the vast majority of experimental efforts within biology (and particularly molecular biology) over the last sixty years. Some of the reasons for this extraordinary success are practical. They are relatively small in size, highly tractable, and have low maintenance costs. They also possess biological traits — such as the transparent skin of zebra-fish, which enable the observation of developmental processes without constant invasive interventions — that make them particularly useful for experimental research. In the words of Adele Clarke and Joan Fujimura, they are "the right tools for the job." Most important for my present purposes, however, are the scientific expectations linked to focusing research on model organisms. It is typically assumed that insights obtained on their functioning and structure will foster the understanding of other species, ranging from relatively similar organisms (Arabidopsis generating insights into crop species, for instance) all the way to humans (as most obviously in the case of mice, which are routinely used as models for human diseases). This does not necessarily mean that model organisms are intrinsically better representations of biological phenomena than other species. Rather, the main reason for the success of these organisms lies in the way in which research on them has been managed and directed from the outset, particularly the interdisciplinary ambitions and collaborative ethos underlying their adoption as laboratory materials.
(Continues...)
Excerpted from Data-Centric Biology by Sabina Leonelli. Copyright © 2016 The University of Chicago. Excerpted by permission of The University of Chicago Press.
All rights reserved. No part of this excerpt may be reproduced or reprinted without permission in writing from the publisher.
Excerpts are provided by Dial-A-Book Inc. solely for the personal use of visitors to this web site.
Table of Contents
IntroductionPart One: Data Journeys
1 Making Data Travel: Technology and Expertise
1.1 The Rise of Online Databases in Biology
1.2 Packaging Data for Travel
1.3 The Emerging Power of Database Curators
1.4 Data Journeys and Other Metaphors of Travel
2 Managing Data Journeys: Social Structures
2.1 The Institutionalization of Data Packaging
2.2 Centralization, Dissent, and Epistemic Diversity
2.3 Open Data as Global Commodities
2.4 Valuing Data
Part Two: Data-Centric Science
3 What Counts as Data? 3.1 Data in the Philosophy of Science
3.2 A Relational Framework
3.3 The Nonlocality of Data
3.4 Packaging and Modeling
4 What Counts as Experiment?
4.1 Capturing Embodied Knowledge
4.2 When Standards Are Not Enough
4.3 Distributed Reasoning in Data Journeys
4.4 Dreams of Automation and Replicability
5 What Counts as Theory?
5.1 Classifying Data for Travel
5.2 Bio-Ontologies as Classificatory Theories
5.3 The Epistemic Role of Classification
5.4 Features of Classificatory Theories
5.5 Theory in Data-Centric Science
Part Three: Implications for Biology and Philosophy
6 Researching Life in the Digital Age
6.1 Varieties of Data Integration, Different Ways to Understand Organisms
6.2 The Impact of Data Centrism: Dangers and Exclusions
6.3 The Novelty of Data Centrism: Opportunities and Future Developments
7 Handling Data to Produce Knowledge
7.1 Problematizing Context
7.2 From Contexts to Situations
7.3 Situating Data in the Digital Age
Conclusion
Acknowledgments
Notes
Bibliography
Index