Addressing the current tension within the artificial intelligence community between advocates of powerful symbolic representations that lack efficient learning procedures and advocates of relatively simple learning procedures that lack the ability to represent complex structures effectively.
The six contributions in Connectionist Symbol Processing address the current tension within the artificial intelligence community between advocates of powerful symbolic representations that lack efficient learning procedures and advocates of relatively simple learning procedures that lack the ability to represent complex structures effectively. The authors seek to extend the representational power of connectionist networks without abandoning the automatic learning that makes these networks interesting.Aware of the huge gap that needs to be bridged, the authors intend their contributions to be viewed as exploratory steps in the direction of greater representational power for neural networks. If successful, this research could make it possible to combine robust general purpose learning procedures and inherent representations of artificial intelligence--a synthesis that could lead to new insights into both representation and learning.
Addressing the current tension within the artificial intelligence community between advocates of powerful symbolic representations that lack efficient learning procedures and advocates of relatively simple learning procedures that lack the ability to represent complex structures effectively.
The six contributions in Connectionist Symbol Processing address the current tension within the artificial intelligence community between advocates of powerful symbolic representations that lack efficient learning procedures and advocates of relatively simple learning procedures that lack the ability to represent complex structures effectively. The authors seek to extend the representational power of connectionist networks without abandoning the automatic learning that makes these networks interesting.Aware of the huge gap that needs to be bridged, the authors intend their contributions to be viewed as exploratory steps in the direction of greater representational power for neural networks. If successful, this research could make it possible to combine robust general purpose learning procedures and inherent representations of artificial intelligence--a synthesis that could lead to new insights into both representation and learning.
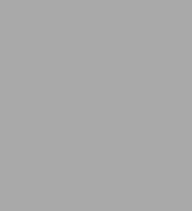
Connectionist Symbol Processing
270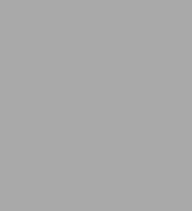
Connectionist Symbol Processing
270Paperback(Mit Press ed.)
Product Details
ISBN-13: | 9780262581066 |
---|---|
Publisher: | MIT Press |
Publication date: | 10/17/1991 |
Series: | Special Issues of Artificial Intelligence |
Edition description: | Mit Press ed. |
Pages: | 270 |
Sales rank: | 532,583 |
Product dimensions: | 8.50(w) x 11.00(h) x 0.50(d) |
Age Range: | 18 Years |