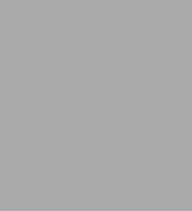
Bias and Causation: Models and Judgment for Valid Comparisons
376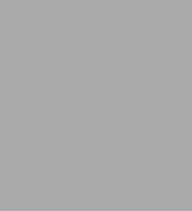
Bias and Causation: Models and Judgment for Valid Comparisons
376eBook
Available on Compatible NOOK devices, the free NOOK App and in My Digital Library.
Related collections and offers
Overview
Do cell phones cause cancer? Can a new curriculum increase student achievement? Determining what the real causes of such problems are, and how powerful their effects may be, are central issues in research across various fields of study. Some researchers are highly skeptical of drawing causal conclusions except in tightly controlled randomized experiments, while others discount the threats posed by different sources of bias, even in less rigorous observational studies. Bias and Causation presents a complete treatment of the subject, organizing and clarifying the diverse types of biases into a conceptual framework. The book treats various sources of bias in comparative studies—both randomized and observational—and offers guidance on how they should be addressed by researchers.
Utilizing a relatively simple mathematical approach, the author develops a theory of bias that outlines the essential nature of the problem and identifies the various sources of bias that are encountered in modern research. The book begins with an introduction to the study of causal inference and the related concepts and terminology. Next, an overview is provided of the methodological issues at the core of the difficulties posed by bias. Subsequent chapters explain the concepts of selection bias, confounding, intermediate causal factors, and information bias along with the distortion of a causal effect that can result when the exposure and/or the outcome is measured with error. The book concludes with a new classification of twenty general sources of bias and practical advice on how mathematical modeling and expert judgment can be combined to achieve the most credible causal conclusions.
Throughout the book, examples from the fields of medicine, public policy, and education are incorporated into the presentation of various topics. In addition, six detailed case studies illustrate concrete examples of the significance of biases in everyday research.
Requiring only a basic understanding of statistics and probability theory, Bias and Causation is an excellent supplement for courses on research methods and applied statistics at the upper-undergraduate and graduate level. It is also a valuable reference for practicing researchers and methodologists in various fields of study who work with statistical data.
This book was selected as the 2011 Ziegel Prize Winner in Technometrics for the best book reviewed by the journal.
It is also the winner of the 2010 PROSE Award for Mathematics from The American Publishers Awards for Professional and Scholarly Excellence
Product Details
ISBN-13: | 9781118058206 |
---|---|
Publisher: | Wiley |
Publication date: | 01/06/2011 |
Series: | Wiley Series in Probability and Statistics , #885 |
Sold by: | JOHN WILEY & SONS |
Format: | eBook |
Pages: | 376 |
File size: | 3 MB |
About the Author
Read an Excerpt
Table of Contents
Preface xi
1 What Is Bias? 1
1.1 Apples and Oranges 2
1.2 Statistics vs. Causation 3
1.3 Bias in the Real World 6
Guidepost 1 23
2 Causality and Comparative Studies 24
2.1 Bias and Causation 24
2.2 Causality and Counterfactuals 26
2.3 Why Counterfactuals? 32
2.4 Causal Effects 33
2.5 Empirical Effects 38
Guidepost 2 46
3 Estimating Causal Effects 47
3.1 External Validity 48
3.2 Measures of Empirical Effects 50
3.3 Difference of Means 52
3.4 Risk Difference and Risk Ratio 55
3.5 Potential Outcomes 57
3.6 Time-Dependent Outcomes 60
3.7 Intermediate Variables 63
3.8 Measurement of Exposure 64
3.9 Measurement of the Outcome Value 68
3.10 Confounding Bias 70
Guidepost 3 71
4 Varieties of Bias 72
4.1 Research Designs and Bias 73
4.2 Bias in Biomedical Research 81
4.3 Bias in Social Science Research 85
4.4 Sources of Bias: A Proposed Taxonomy 90
Guidepost 4 92
5 Selection Bias 93
5.1 Selection Processes and Bias 93
5.2 Traditional Selection Model: Dichotomous Outcome 100
5.3 Causal Selection Model: Dichotomous Outcome 102
5.4 Randomized Experiments 104
5.5 Observational Cohort Studies 108
5.6 Traditional Selection Model: Numerical Outcome 111
5.7 Causal Selection Model: Numerical Outcome 114
Guidepost 5 121
Appendix 122
6 Confounding: An Enigma? 126
6.1 What is the Real Problem? 127
6.2 Confounding and Extraneous Causes 128
6.3 Confounding and Statistical Control 131
6.4 Confounding and Comparability 137
6.5 Confounding and the Assignment Mechanism 139
6.6 Confounding and Model Specification 141
Guidepost 6 144
7 Confounding: Essence, Correction, and Detection 145
7.1 Essence: The Nature of Confounding 146
7.2 Correction: Statistical Control for Confounding 172
7.3 Detection: Adequacy of Statistical Adjustment 180
Guidepost 7 191
Appendix 192
8 Intermediate Causal Factors 195
8.1 Direct and Indirect Effects 195
8.2 Principal Stratification 200
8.3 Noncompliance 209
8.4 Attrition 214
Guidepost 8 216
9 Information Bias 217
9.1 Basic Concepts 218
9.2 Classical Measurement Model: Dichotomous Outcome 223
9.3 Causal Measurement Model: Dichotomous Outcome 230
9.4 Classical Measurement Model: Numerical Outcome 239
9.5 Causal Measurement Model: Numerical Outcome 242
9.6 Covariates Measured with Error 246
Guidepost 9 250
10 Sources of Bias 252
10.1 Sampling 254
10.2 Assignment 260
10.3 Adherence 266
10.4 Exposure Ascertainment 269
10.5 Outcome Measurement 273
Guidepost 10 277
11 Contending with Bias 279
11.1 Conventional Solutions 280
11.2 Standard Statistical Paradigm 286
11.3 Toward a Broader Perspective 288
11.4 Real-World Bias Revisited 293
11.5 Statistics and Causation 303
Glossary 309
Bibliography 321
Index 340
What People are Saying About This
"The book combines a useful synthesis of the literature with an original working through of issues related to bias and causal inference. Anyone with a sustained interest in this topic will find the book worth reading." (Journal of Educational and Behavioral Statistics, May 2012)
"...the book provides a unified framework for understanding issues of causal inference discussed differently across disciplines...the book will also be of substantial interest to methodologically minded readers working within specific disciplines but interested in methodological literature from other disciplines." (Journal of Educational and Behavioral Statistics, May 2012)
"The book covers almost all the relevant biases that can be present when designing and analyzing treatment effects in comparative studies." (Journal of Biopharmaceutical Statistics, January 2011)"A consultant who specializes in applying statistics to various business and legal issues, Weisberg explains approaches to bias and causal inference, a realm statisticians have avoided until recently because it requires intuitive skills beyond the pale of mathematics. He writes for practicing researchers and methodologists and for students with a reasonably solid grounding in basic statistics and research methods." (SciTech Book News, December 2010)