5
1
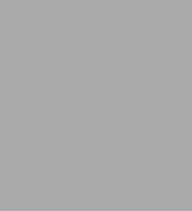
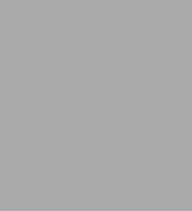
eBookThird Edition (Third Edition)
$75.99
$101.00
Save 25%
Current price is $75.99, Original price is $101. You Save 25%.
Available on Compatible NOOK devices, the free NOOK App and in My Digital Library.
WANT A NOOK?
Explore Now
Related collections and offers
75.99
In Stock
Overview
Rebecca M. Warner’s bestselling Applied Statistics: From Bivariate Through Multivariate Techniques has been split into two volumes for ease of use over a two-course sequence. Applied Statistics I: Basic Bivariate Techniques, Third Edition is an introductory statistics text based on chapters from the first half of the original book.
The author′s contemporary approach reflects current thinking in the field, with its coverage of the "new statistics" and reproducibility in research. Her in-depth presentation of introductory statistics follows a consistent chapter format, includes some simple hand-calculations along with detailed instructions for SPSS, and helps students understand statistics in the context of real-world research through interesting examples. Datasets are provided on an accompanying website.
Bundle and Save
Applied Statistics I + Applied Statistics II: Basic Bivariate Techniques, Third Edition
Bundle Volume I and II ISBN: 978-1-0718-1337-9
An R Companion for Applied Statistics I: Basic Bivariate Techniques + Applied Statistics I
Bundle ISBN: 978-1-0718-1325-6
The author′s contemporary approach reflects current thinking in the field, with its coverage of the "new statistics" and reproducibility in research. Her in-depth presentation of introductory statistics follows a consistent chapter format, includes some simple hand-calculations along with detailed instructions for SPSS, and helps students understand statistics in the context of real-world research through interesting examples. Datasets are provided on an accompanying website.
Bundle and Save
Applied Statistics I + Applied Statistics II: Basic Bivariate Techniques, Third Edition
Bundle Volume I and II ISBN: 978-1-0718-1337-9
An R Companion for Applied Statistics I: Basic Bivariate Techniques + Applied Statistics I
Bundle ISBN: 978-1-0718-1325-6
Product Details
ISBN-13: | 9781506352824 |
---|---|
Publisher: | SAGE Publications |
Publication date: | 01/14/2020 |
Sold by: | Barnes & Noble |
Format: | eBook |
Pages: | 648 |
Sales rank: | 960,773 |
File size: | 86 MB |
Note: | This product may take a few minutes to download. |
About the Author
Rebecca M. Warner received a B.A. from Carnegie-Mellon University in Social Relations in 1973 and a Ph.D. in Social Psychology from Harvard in 1978. She has taught statistics for more than 25 years: from Introductory and Intermediate Statistics to advanced topics seminars in Multivariate Statistics, Structural Equation Modeling, and Time Series Analysis. She is currently a Full Professor in the Department of Psychology at the University of New Hampshire. She is a Fellow in the Association for Psychological Science and a member of the American Psychological Association, the International Association for Relationships Research, the Society of Experimental Social Psychology, and the Society for Personality and Social Psychology. She has consulted on statistics and data management for the World Health Organization in Geneva and served as a visiting faculty member at Shandong Medical University in China.
Table of Contents
PrefaceAcknowledgments
About the Author
1. Evaluating Numerical Information
Introduction
Guidelines for Numeracy
Source Credibility
Message Content
Evaluating Generalizability
Making Causal Claims
Quality Control Mechanisms in Science
Biases of Information Consumers
Ethical Issues in Data Collection and Analysis
Lying with Graphs and Statistics
Degrees of Belief
Summary
2. Basic Research Concepts
Introduction
Types of Variables
Independent and Dependent Variables
Typical Research Questions
Conditions for Causal Inference
Experimental Research Design
Nonexperimental Research Design
Quasi-Experimental Research Designs
Other Issues in Design and Analysis
Choice of Statistical Analysis (Preview)
Populations and Samples: Ideal Versus Actual Situations
Common Problems in Interpretation of Results
Appendix 2A: More About Levels of Measurement
Appendix 2B: Justification for the Use of Likert and Other Rating Scales as Quantitative Variables (in Some Situations)
3. Frequency Distribution Tables
Introduction
Use of Frequency Tables for Data Screening
Frequency Tables for Categorical Variables
Elements of Frequency Tables
Using SPSS to Obtain a Frequency Table
Mode, Impossible Score Values, and Missing Values
Reporting Data Screening for Categorical Variables
Frequency Tables for Quantitative Variables
Frequency Tables for Categorical Versus Quantitative Variables
Reporting Data Screening for Quantitative Variables
What We Hope to See in Frequency Tables for Categorical Variables
What We Hope to See in Frequency Tables for Quantitative Variables
Summary
Appendix 3A: Getting Started in IBM SPSS® Version 25
Appendix 3B: Missing Values in Frequency Tables
Appendix 3C: Dividing Scores Into Groups or Bins
4. Descriptive Statistics
Introduction
Questions about Quantitative Variables
Notation
Sample Median
Sample Mean (M)
An Important Characteristic of M: The Sum of Deviations From M = 0
Disadvantage of M: It is Not Robust Against Influence of Extreme Scores
Behavior of Mean, Median, and Mode in Common Real-World Situations
Choosing Among Mean, Median, and Mode
Using SPSS to Obtain Descriptive Statistics for a Quantitative Variable
Minimum, Maximum, and Range: Variation among Scores
The Sample Variance s2
Sample Standard Deviation (s or SD)
How a Standard Deviation Describes Variation Among Scores in a Frequency Table
Why Is There Variance?
Reports of Descriptive Statistics in Journal Articles
Additional Issues in Reporting Descriptive Statistics
Summary
Appendix 4A: Order of Arithmetic Operations
Appendix 4B: Rounding
5. Graphs: Bar Charts, Histograms, and Boxplots
Introduction
Pie Charts for Categorical Variables
Bar Charts for Frequencies of Categorical Variables
Good Practice for Construction of Bar Charts
Deceptive Bar Graphs
Histograms for Quantitative Variables
Obtaining a Histogram Using SPSS
Describing and Sketching Bell-Shaped Distributions
Good Practices in Setting up Histograms
Boxplot (Box and Whiskers Plot)
Telling Stories About Distributions
Uses of Graphs in Actual Research
Data Screening: Separate Bar Charts or Histograms for Groups
Use of Bar Charts to Represent Group Means
Other Examples
Summary
6. The Normal Distribution and z Scores
Introduction
Locations of Individual Scores in Normal Distributions
Standardized or z Scores
Converting z Scores Back Into X Units
Understanding Values of z
Qualitative Description of Normal Distribution Shape
More Precise Description of Normal Distribution Shape
Areas Under the Normal Distribution Curve Can Be Interpreted as Probabilities
Reading Tables of Areas for the Standard Normal Distribution
Dividing the Normal Distribution Into Three Regions: Lower Tail, Middle, Upper Tail
Outliers Relative to a Normal Distribution
Summary of First Part of Chapter
Why We Assess Distribution Shape
Departure from Normality: Skewness
Another Departure from Normality: Kurtosis
Overall Normality
Practical Recommendations for Preliminary Data Screening and Descriptions of Scores for Quantitative Variables
Reporting Information About Distribution Shape, Missing Values, Outliers, and Descriptive Statistics for Quantitative Variables
Summary
Appendix 6A: The Mathematics of the Normal Distribution
Appendix 6B: How to Select and Remove Outliers in SPSS
Appendix 6C: Quantitative Assessments of Departure From Normality
Appendix 6D: Why Are Some Real-World Variables Approximately
7. Sampling Error and Confidence Intervals
Descriptive Versus Inferential Uses of Statistics
Notation for Samples Versus Populations
Sampling Error and the Sampling Distribution for Values of M
Prediction Error
Sample Versus Population (Revisited)
The Central Limit Theorem: Characteristics of the Sampling Distribution of M
Factors That Influence Population Standard Error (sM)
Effect of N on Value of the Population Standard Error
Describing the Location of a Single Outcome for M Relative to Population Sampling Distribution (Setting Up a z Ratio)
What We Do When s Is Unknown
The Family of t Distributions
Tables for t Distributions
Using Sampling Error to Set Up a Confidence Interval
How to Interpret a Confidence Interval
Empirical Example: Confidence Interval for Body Temperature
Other Applications for Confidence Intervals
Error Bars in Graphs of Group Means
Summary
8. The One-Sample t test: Introduction to Statistical Significance Tests
Introduction
Significance Tests as Yes/No Questions About Proposed Values of Population Means
Stating a Null Hypothesis
Selecting an Alternative Hypothesis
The One-Sample t Test
Choosing an Alpha (a) Level
Specifying Reject Regions on the Basis of a, Halt, and df
Questions for the One-Sample t Test
Assumptions for the Use of the One-Sample t Test
Rules for the Use of NHST
First Analysis of Mean Driving Speed Data (Using a Nondirectional Test)
SPSS Analysis: One-Sample t Test for Mean Driving Speed (Using a Nondirectional or Two-Tailed Test)
“Exact” p Values
Reporting Results for a Two-tailed One-Sample t Test
Second Analysis of Driving Speed Data Using a One-Tailed or Directional Test
Reporting Results for a One-tailed One-Sample t Test
Advantages and Disadvantages of One-Tailed Tests
Traditional NHST Versus New Statistics Recommendations
Things You Should Not Say About p Values
Summary
9. Issues in Significance Tests: Effect Size, Statistical Power, and Decision Errors
Beyond p Values
Cohen’s d: An Effect Size Index
Factors that Affect the Size of t Ratios
Statistical Significance Versus Practical Importance
Statistical Power
Type I and Type II Decision Errors
Meanings of “Error”
Use of NHST in Exploratory Versus Confirmatory Research
Inflated Risk for Type I Decision Error for Multiple Tests
Interpretation of Null Outcomes
Interpretation of Statistically Significant Outcomes
Understanding Past Research
Planning Future Research
Guidelines for Reporting Results
What You Cannot Say
Summary
Appendix 9A: Further Explanation of Statistical Power
10. Bivariate Pearson Correlation
Research Situations Where Pearson’s r Is Used
Correlation and Causal Inference
How Sign and Magnitude of r Describe an X, Y Relationship
Setting Up Scatterplots
Most Associations Are Not Perfect
Different Situations in Which r = .00
Assumptions for Use of Pearson’s r
Preliminary Data Screening for Pearson’s r
Effect of Extreme Bivariate Outliers
Research Example
Data Screening for Research Example
Computation of Pearson’s r
How Computation of Correlation Is Related to Pattern of Data Points in the Scatterplot
Testing the Hypothesis That p0 = 0
Reporting Many Correlations and Inflated Risk for Type I Error
Obtaining Confidence Intervals for Correlations
Pearson’s r and r2 as Effect Sizes and Partition of Variance
Statistical Power and Sample Size for Correlation Studies
Interpretation of Outcomes for Pearson’s r
SPSS Example: Relationship Survey
Results Sections for One and Several Pearson’s r Values
Reasons to Be Skeptical of Correlations
Summary
Appendix 10A: Nonparametric Alternatives to Pearson’s r
Appendix 10B: Setting Up a 95% CI for Pearson’s r by Hand
Appendix 10C: Testing Significance of Differences Between Correlations
Appendix 10D: Some Factors That Artifactually Influence Magnitude of r
Appendix 10E: Analysis of Nonlinear Relationships
Appendix 10F: Alternative Formula to Compute Pearson’s r
11. Bivariate Regression
Research Situations Where Bivariate Regression Is Used
New Information Provided by Regression
Regression Equations and Lines
Two Versions of Regression Equations
Steps in Regression Analysis
Preliminary Data Screening
Formulas for Bivariate Regression Coefficients
Statistical Significance Tests for Bivariate Regression
Confidence Intervals for Regression Coefficients
Effect Size and Statistical Power
Empirical Example Using SPSS: Salary Data
SPSS Output: Salary Data
Results Section: Hypothetical Salary Data
Plotting the Regression Line: Salary Data
Using a Regression Equation to Predict Score for Individual (Joe’s Heart Rate Data)
Partition of Sums of Squares in Bivariate Regression
Why Is There Variance (Revisited)?
Issues in Planning a Bivariate Regression Study
Plotting Residuals
Standard Error of the Estimate
Summary
Appendix 11A: Review: How to Graph a Line From Two Points Obtained From an Equation
Appendix 11B: OLS Derivation of Equation for Regression Coefficients
Appendix 11C: Alternative Formula for Computation of Slope
Appendix 11D: Fully Worked Example: Deviations and SS
12. The Independent-Samples t Test
Research Situations Where the Independent-Samples t Test Is Used
Hypothetical Research Example
Assumptions for Use of Independent-Samples t Test
Preliminary Data Screening: Evaluating Violations of Assumptions and Getting to Know Your Data
Computation of Independent-Samples t Test
Statistical Significance of Independent-Samples t Test
Confidence Interval Around M1 – M2
SPSS Commands for Independent-Samples t Test
SPSS Output for Independent-Samples t Test
Effect Size Indexes for t
Factors that Influence the Size of t
Results Section
Graphing Results: Means and CIs
Decisions About Sample Size for the Independent-Samples t Test
Issues in Designing a Study
Summary
Appendix 12A: A Nonparametric Alternative to the Independent-Samples t Test
13. One-Way Between-Subjects Analysis of Variance
Research Situations Where One-Way ANOVA Is Used
Questions in One-Way Between-S ANOVA
Hypothetical Research Example
Assumptions and Data Screening for One-Way ANOVA
Computations for One-Way Between-S ANOVA
Patterns of Scores and Magnitudes of SSbetween and SSwithin
Confidence Intervals for Group Means
Effect Sizes for One-Way Between-S ANOVA
Statistical Power Analysis for One-Way Between-S ANOVA
Planned Contrasts
Post Hoc or “Protected” Tests
One-Way Between-S ANOVA in SPSS
Output From SPSS for One-Way Between-S ANOVA
Reporting Results From One-Way Between-S ANOVA
Issues in Planning a Study
Summary
Appendix 13A: ANOVA Model and Division of Scores Into Components
Appendix 13B: Expected Value of F When H0 Is True
Appendix 13C: Comparison of ANOVA and t Test
Appendix 13D: Nonparametric Alternative to One-Way Between-S ANOVA: Independent-Samples Kruskal-Wallis Test
14. Paired-Samples t Test
Independent- Versus Paired-Samples Designs
Between-S and Within-S or Paired-Groups Designs
Types of Paired Samples
Hypothetical Study: Effects of Stress on Heart Rate
Review: Data Organization for Independent Samples
New: Data Organization for Paired Samples
A First Look at Repeated-Measures Data
Calculation of Difference (d) Scores
Null Hypothesis for Paired-Samples t Test
Assumptions for Paired-Samples t Test
Formulas for Paired-Samples t Test
SPSS Paired-Samples t Test Procedure
Comparison Between Results for Independent-Samples and Paired-Samples t Tests
Effect Size and Power
Some Design Problems in Repeated-Measures Analyses
Results for Paired-Samples t Test: Stress and Heart Rate
Further Evaluation of Assumptions
Summary
Appendix 14A: Nonparametric Alternative to Paired-Samples t: Wilcoxon Signed Rank Test
15. One-Way Repeated-Measures Analysis of Variance
Introduction
Null Hypothesis for Repeated-Measures ANOVA
Preliminary Assessment of Repeated-Measures Data
Computations for One-Way Repeated-Measures ANOVA
Use of SPSS Reliability Procedure for One-Way Repeated-Measures ANOVA
Partition of SS in Between-S Versus Within-S ANOVA
Assumptions for Repeated-Measures ANOVA
Choices of Contrasts in GLM Repeated Measures
SPSS GLM Procedure for Repeated-Measures ANOVA
Output of GLM Repeated-Measures ANOVA
Paired-Samples t Tests as Follow-Up
Results
Effect Size
Statistical Power
Counterbalancing in Repeated-Measures Studies
More Complex Designs
Summary
Appendix 15A: Test for Person-by-Treatment Interaction
Appendix 15B: Nonparametric Analysis for Repeated Measures (Friedman Test)
16. Factorial Analysis of Variance
Research Situations Where Factorial Design Is Used
Questions in Factorial ANOVA
Null Hypotheses in Factorial ANOVA
Screening for Violations of Assumptions
Hypothetical Research Situation
Computations for Between-S Factorial ANOVA
Computation of SS and df in Two-Way Factorial ANOVA
Effect Size Estimates for Factorial ANOVA
Statistical Power
Follow-Up Tests
Factorial ANOVA Using the SPSS GLM Procedure
SPSS Output
Results
Design Decisions and Magnitudes of SS Terms
Summary
Appendix 16A: Fixed Versus Random Factors
Appendix 16B: Weighted Versus Unweighted Means
Appendix 16C: Unequal Cell n’s in Factorial ANOVA: Computing Adjusted Sums of Squares
Appendix 16D: Model for Factorial ANOVA
Appendix 16E: Computation of Sums of Squares by Hand
17. Chi-Square Analysis of Contingency Tables
Evaluating Association Between Two Categorical Variables
First Example: Contingency Tables for Titanic Data
What Is Contingency?
Conditional and Unconditional Probabilities
Null Hypothesis for Contingency Table Analysis
Second Empirical Example: Dog Ownership Data
Preliminary Examination of Dog Ownership Data
Expected Cell Frequencies If H0 Is True
Computation of Chi Squared Significance Test
Evaluation of Statistical Significance of x2
Effect Sizes for Chi Squared
Chi Squared Example Using SPSS
Output From Crosstabs Procedure
Reporting Results
Assumptions and Data Screening for Contingency Tables
Other Measures of Association for Contingency Tables
Summary
Appendix 17A: Margin of Error for Percentages in Surveys
Appendix 17B: Contingency Tables With Repeated Measures: McNemar Test
Appendix 17C: Fisher Exact Test
Appendix 17D: How Marginal Distributions for X and Y Constrain Maximum Value of f
Appendix 17E: Other Uses of x2
18. Selection of Bivariate Analyses and Review of Key Concepts
Selecting Appropriate Bivariate Analyses
Types of Independent and Dependent Variables (Categorical Versus Quantitative
Parametric Versus Nonparametric Analyses
Comparisons of Means or Medians Across Groups (Categorical IV and Quantitative DV)
Problems With Selective Reporting of Evidence and Analyses
Limitations of Statistical Significance Tests and p Values
Statistical Versus Practical Significance
Generalizability Issues
Causal Inference
Results Sections
Beyond Bivariate Analyses: Adding Variables
Some Multivariable or Multivariate Analyses
Degree of Belief
Appendices
Appendix A: Proportions of Area Under a Standard Normal Curve
Appendix B: Critical Values for t Distribution
Appendix C: Critical Values of F
Appendix D: Critical Values of Chi-Square
Appendix E: Critical Values of the Pearson Correlation Coefficient
Appendix F: Critical Values of the Studentized Range Statistic
Appendix G: Transformation of r (Pearson Correlation) to Fisher’s Z
Glossary
References
Index
From the B&N Reads Blog
Page 1 of