Providing a practical introduction to state space methods as applied to unobserved components time series models, also known as structural time series models, this book introduces time series analysis using state space methodology to readers who are neither familiar with time series analysis, nor with state space methods. The only background required in order to understand the material presented in the book is a basic knowledge of classical linear regression models, of which a brief review is provided to refresh the reader's knowledge. Also, a few sections assume familiarity with matrix algebra, however, these sections may be skipped without losing the flow of the exposition. The book offers a step by step approach to the analysis of the salient features in time series such as the trend, seasonal, and irregular components. Practical problems such as forecasting and missing values are treated in some detail. This useful book will appeal to practitioners and researchers who use time series on a daily basis in areas such as the social sciences, quantitative history, biology and medicine. It also serves as an accompanying textbook for a basic time series course in econometrics and statistics, typically at an advanced undergraduate level or graduate level.
1101393647
An Introduction to State Space Time Series Analysis
Providing a practical introduction to state space methods as applied to unobserved components time series models, also known as structural time series models, this book introduces time series analysis using state space methodology to readers who are neither familiar with time series analysis, nor with state space methods. The only background required in order to understand the material presented in the book is a basic knowledge of classical linear regression models, of which a brief review is provided to refresh the reader's knowledge. Also, a few sections assume familiarity with matrix algebra, however, these sections may be skipped without losing the flow of the exposition. The book offers a step by step approach to the analysis of the salient features in time series such as the trend, seasonal, and irregular components. Practical problems such as forecasting and missing values are treated in some detail. This useful book will appeal to practitioners and researchers who use time series on a daily basis in areas such as the social sciences, quantitative history, biology and medicine. It also serves as an accompanying textbook for a basic time series course in econometrics and statistics, typically at an advanced undergraduate level or graduate level.
63.99
In Stock
5
1
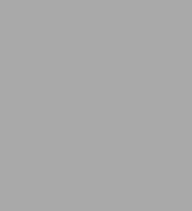
An Introduction to State Space Time Series Analysis
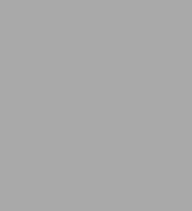
An Introduction to State Space Time Series Analysis
eBook
$63.99
$84.99
Save 25%
Current price is $63.99, Original price is $84.99. You Save 25%.
Related collections and offers
63.99
In Stock
Product Details
ISBN-13: | 9780191607806 |
---|---|
Publisher: | OUP Oxford |
Publication date: | 07/19/2007 |
Series: | Practical Econometrics |
Sold by: | Barnes & Noble |
Format: | eBook |
File size: | 12 MB |
Note: | This product may take a few minutes to download. |
About the Author
From the B&N Reads Blog