Algorithmic Learning in a Random World
Algorithmic Learning in a Random World describes recent theoretical and experimental developments in building computable approximations to Kolmogorov's algorithmic notion of randomness. Based on these approximations, a new set of machine learning algorithms have been developed that can be used to make predictions and to estimate their confidence and credibility in high-dimensional spaces under the usual assumption that the data are independent and identically distributed (assumption of randomness). Another aim of this unique monograph is to outline some limits of predictions: The approach based on algorithmic theory of randomness allows for the proof of impossibility of prediction in certain situations. The book describes how several important machine learning problems, such as density estimation in high-dimensional spaces, cannot be solved if the only assumption is randomness.
1141455304
Algorithmic Learning in a Random World
Algorithmic Learning in a Random World describes recent theoretical and experimental developments in building computable approximations to Kolmogorov's algorithmic notion of randomness. Based on these approximations, a new set of machine learning algorithms have been developed that can be used to make predictions and to estimate their confidence and credibility in high-dimensional spaces under the usual assumption that the data are independent and identically distributed (assumption of randomness). Another aim of this unique monograph is to outline some limits of predictions: The approach based on algorithmic theory of randomness allows for the proof of impossibility of prediction in certain situations. The book describes how several important machine learning problems, such as density estimation in high-dimensional spaces, cannot be solved if the only assumption is randomness.
199.99
In Stock
5
1
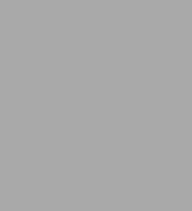
Algorithmic Learning in a Random World
476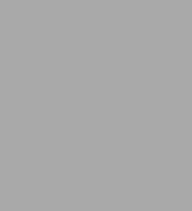
Algorithmic Learning in a Random World
476Hardcover(2nd ed. 2022)
$199.99
199.99
In Stock
Product Details
ISBN-13: | 9783031066481 |
---|---|
Publisher: | Springer International Publishing |
Publication date: | 12/14/2022 |
Edition description: | 2nd ed. 2022 |
Pages: | 476 |
Product dimensions: | 6.10(w) x 9.25(h) x (d) |
About the Author
From the B&N Reads Blog