A Primer in Econometric Theory available in Hardcover
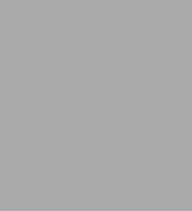
- ISBN-10:
- 0262034905
- ISBN-13:
- 9780262034906
- Pub. Date:
- 08/05/2016
- Publisher:
- MIT Press
- ISBN-10:
- 0262034905
- ISBN-13:
- 9780262034906
- Pub. Date:
- 08/05/2016
- Publisher:
- MIT Press
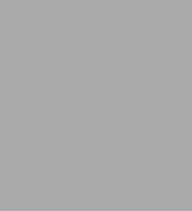
Hardcover
Buy New
$60.00Buy Used
$41.29-
-
SHIP THIS ITEM
Temporarily Out of Stock Online
Please check back later for updated availability.
-
Overview
This book offers a cogent and concise treatment of econometric theory and methods along with the underlying ideas from statistics, probability theory, and linear algebra. It emphasizes foundations and general principles, but also features many solved exercises, worked examples, and code listings. After mastering the material presented, readers will be ready to take on more advanced work in different areas of quantitative economics and to understand papers from the econometrics literature. The book can be used in graduate-level courses on foundational aspects of econometrics or on fundamental statistical principles. It will also be a valuable reference for independent study.
One distinctive aspect of the text is its integration of traditional topics from statistics and econometrics with modern ideas from data science and machine learning; readers will encounter ideas that are driving the current development of statistics and increasingly filtering into econometric methodology. The text treats programming not only as a way to work with data but also as a technique for building intuition via simulation. Many proofs are followed by a simulation that shows the theory in action. As a primer, the book offers readers an entry point into the field, allowing them to see econometrics as a whole rather than as a profusion of apparently unrelated ideas.
Product Details
ISBN-13: | 9780262034906 |
---|---|
Publisher: | MIT Press |
Publication date: | 08/05/2016 |
Series: | The MIT Press |
Edition description: | New Edition |
Pages: | 448 |
Product dimensions: | 7.25(w) x 9.31(h) x 1.13(d) |
Age Range: | 18 Years |
About the Author
Table of Contents
Preface xv
Common Symbols xvii
1 Introduction 1
1.1 The Nature of Econometrics 1
1.2 Data versus Theory 3
1.3 Comments on the Literature 5
1.4 Further Reading 5
I Background 7
2 Vector Spaces 9
2.1 Vectors and Vector Space 9
2.1.1 Vectors 9
2.1.2 Linear Combinations and Span 14
2.1.3 Linear Independence 17
2.1.4 Linear Subspaces 20
2.1.5 Bases and Dimension 21
2.1.6 Linear Maps 23
2.1.7 Linear Independence and Bijections 25
2.2 Orthogonality 27
2.2.1 Definition and Basic Properties 27
2.2.2 The Orthogonal Projection Theorem 29
2.2.3 Projection as a Mapping 30
2.2.4 The Residual Projection 32
2.3 Further Reading 34
2.4 Exercises 35
2.4.1 Solutions to Selected Exercises 37
3 Linear Algebra and Matrices 45
3.1 Matrices and Linear Equations 45
3.1.1 Basic Definitions 45
3.1.2 Matrices as Maps 48
3.1.3 Square Matrices and Invertibility 50
3.1.4 Determinants 52
3.2 Properties of Matrices 53
3.2.1 Diagonal and Triangular Matrices 53
3.2.2 Trace, Transpose, and Symmetry 54
3.2.3 Eigenvalues and Eigenvectors 55
3.2.4 Quadratic Forms 57
3.3 Projection and Decomposition 60
3.3.1 Projection Matrices 60
3.3.2 Over determined Systems of Equations 62
3.3.3 QR Decomposition 64
3.3.4 Diagonalization and Spectral Theory 65
3.3.5 Norms and Continuity 67
3.4 Further Reading 70
3.5 Exercises 70
3.5.1 Solutions to Selected Exercises 73
4 Foundations of Probability 79
4.1 Probabilistic Models 79
4.1.1 Sample Spaces and Events 79
4.1.2 Probabilities 83
4.1.3 Random Variables 89
4.1.4 Expectations 93
4.1.5 Moments and Co-Moments 97
4.2 Distributions 99
4.2.1 Defining Distributions on IR 100
4.2.2 Densities and PMFs 103
4.2.3 Integrating with Distributions 108
4.2.4 Distributions of Random Variables 110
4.2.5 Expectations from Distributions 112
4.2.6 Quantile Functions 113
4.3 Further Reading 116
4.4 Exercises 116
4.4.1 Solutions to Selected Exercises 118
5 Modeling Dependence 125
5.1 Random Vectors and Matrices 125
5.1.1 Random Vectors 125
5.1.2 Multivariate Distributions 127
5.1.3 Distributions of Random Vectors 132
5.1.4 Independence 135
5.1.5 Copulas 138
5.1.6 Properties of Named Distributions 140
5.2 Conditioning and Expectation 141
5.2.1 Conditional Distributions 141
5.2.2 The Space L2 142
5.2.3 Projections in L2 145
5.2.4 Measurability 148
5.2.5 Conditional Expectation 150
5.2.6 The Vector Case 153
5.3 Further Reading 154
5.4 Exercises 154
5.4.1 Solutions to Selected Exercises 156
6 Asymptotics 161
6.1 LLN and CLT 161
6.1.1 Convergence of Random Vectors 161
6.1.2 The Law of Large Numbers 163
6.1.3 Convergence in Distribution 165
6.1.4 The Central Limit Theorem 168
6.2 Extensions 169
6.2.1 Convergence of Random Matrices 170
6.2.2 Vector-Valued LLNs and CLTs 171
6.2.3 The Delta Method 173
6.3 Further Reading 174
6.4 Exercises 174
6.4.1 Solutions to Selected Exercises 175
7 Further Topics in Probability 177
7.1 Stochastic Processes 177
7.1.1 Stationarity and Ergodicity 178
7.1.2 Stochastic Recursive Sequences 179
7.2 Markov Processes 184
7.2.1 The Markov Assumption 184
7.2.2 Marginal and Joint Distributions 188
7.2.3 Stationarity of Markov Processes 190
7.2.4 Asymptotics of Markov Processes 193
7.2.5 The Linear Case 196
7.3 Martingales 197
7.3.1 Definitions 197
7.3.2 Martingale Difference LLN and CLT 199
7.4 Simulation 200
7.4.1 Inverse Transforms 200
7.4.2 Markov Chain Monte Carlo 201
7.5 Further Reading 206
7.6 Exercises 206
7.6.1 Solutions to Selected Exercises 207
II Foundations of Statistics 211
8 Estimators 213
8.1 The Estimation Problem 213
8.1.1 Definitions 213
8.1.2 Statistics and Estimators 216
8.1.3 Empirical Distributions 219
8.2 Estimation Principles 222
8.2.1 The Sample Analogue Principle 222
8.2.2 Empirical Risk Minimization 225
8.2.3 The Choice of Hypothesis Space 228
8.3 Some Parametric Methods 233
8.3.1 Maximum Likelihood 234
8.3.2 Maximum Likelihood via ERM 238
8.3.3 The Method of Moments and GMM 239
8.3.4 Bavesian Estimation 241
8.4 Further Reading 244
8.5 Exercises 244
8.5.1 Solutions to Selected Exercises 245
9 Properties of Estimators 247
9.1 Sampling Distributions 247
9.1.1 Estimators as Random Elements 247
9.1.2 Sampling Distributions 248
9.1.3 The Bootstrap 251
9.2 Evaluating Estimators 255
9.2.1 Bias 256
9.2.2 Variance 257
9.2.3 Variance versus Bias 259
9.2.4 Asymptotic Properties 262
9.2.5 Decision Theory 265
9.3 Further Reading 270
9.4 Exercises 270
9.4.1 Solutions to Selected Exercises 272
10 Confidence Intervals and Tests 275
10.1 Confidence Sets 275
10.1.1 Finite Sample Confidence Sets 276
10.1.2 Asymptotic Methods 277
10.1.3 A Nonparametric Example 279
10.2 Hypothesis Tests 280
10.2.1 Constructing Tests 282
10.2.2 Choosing Critical Values 284
10.2.3 Asymptotic Tests 286
10.2.4 Accepting the Null? 289
10.2.5 Statistical Tests in Economics 293
10.3 Further Reading 294
10.4 Exercises 295
10.4.1 Solutions to Selected Exercises 296
III Econometric Models 297
11 Regression 299
11.1 Linear Regression 299
11.1.1 The Setup 299
11.1.2 The Least Squares Estimator 301
11.1.3 Out-of-Sample Fit 304
11.1.4 In-Sample Fit 306
11.2 The Geometry of Least Squares 308
11.2.1 Transformations and Basis Functions 308
11.2.2 The Frisch-Waugh-Lovell Theorem 311
11.2.3 Centered Observations 314
11.3 Further Reading 315
11.4 Exercises 315
11.4.1 Solutions to Selected Exercises 317
12 Ordinary Least Squares 323
12.1 Estimation under OLS 323
12.1.1 Assumptions 323
12.1.2 The OLS Estimators 325
12.1.3 Finite Sample Properties 327
12.1.4 Inference with Normal Errors 331
12.2 Problems and Extensions 338
12.2.1 Nonspherical Errors 338
12.2.2 Bias 340
12.2.3 Instrumental Variables 343
12.2.4 Causality 345
12.3 Further Reading 347
12.4 Exercises 347
12.4.1 Solutions to Selected Exercises 349
13 Large Samples and Dependence 355
13.1 Large Sample Least Squares 355
13.1.1 Setup and Assumptions 355
13.1.2 Consistency 358
13.1.3 Asymptotic Normality of β 359
13.1.4 Large Sample Tests 361
13.2 MLE for Markov Processes 363
13.2.1 The Likelihood Function 363
13.2.2 The Newton-Raphson Algorithm 365
13.3 Further Reading 370
13.4 Exercises 370
13.4.1 Solutions to Selected Exercises 372
14 Regularizatiorv 377
14.1 Nonparametric Density Estimation 377
14.1.1 Introduction 377
14.1.2 Kernel Density Estimation 379
14.1.3 Theory 381
14.1.4 Commentary 386
14.2 Controlling Complexity 386
14.2.1 Ridge Regression 387
14.2.2 Subset Selection and Ridge Regression 389
14.2.3 Bayesian Methods and Regularization 392
14.2.4 Cross-Validation 395
14.3 Further Reading 399
14.4 Exercises 399
14.4.1 Solutions to Selected Exercises 400
IV Appendix 403
15 Appendix 405
15.1 Sets 405
15.1.1 Cartesian Products 408
15.2 Functions 408
15.2.1 Preimage of Sets 411
15.3 Cardinality and Measure 411
15.3.1 Lebesgue Measure and Sets of Measure Zero 412
15.4 Real-Valued Functions 413
15.4.1 Sup and Inf 413
Bibliography 415
Index 425
What People are Saying About This
This important book fills a gap in the existing curriculum by providing a firm foundation in linear algebra, statistics, and coding for students who want to study advanced econometrics. And there's more: it also exposes students to methods of machine learning and computational statistics, offering a broader perspective on modern data analytic techniques.
A Primer in Econometric Theory presents key foundations and supplements them with good examples. It vividly shows the benefits given to us by decades of technical progress in econometrics and computational methods. Stachurski's writing style presents technical arguments in attractive and accessible ways.
When I learned the fundamentals of linear algebra and economic theory as a graduate student, I found most textbooks and courses had an emphasis on theory but very little in terms of software. The emphasis on teaching these ideas through modern programming tools such as Julia, Python, and R not only makes the material far more accessible but also equips the readers of this book for their professional careers. I wish I had such a book when I was in grad school!
John Stachurski's text, A Primer in Econometric Theory is a concise and elegant book that provides a more conceptual introduction to econometrics that coincides well with my own preferred way of teaching the subject to first-year graduate students. It is clear, rigorous, and provides a large number of interesting exercises with solutions. I will use it in my own econometrics teaching and recommend it to complement other applied econometrics books.
When I learned the fundamentals of linear algebra and economic theory as a graduate student, I found most textbooks and courses had an emphasis on theory but very little in terms of software. The emphasis on teaching these ideas through modern programming tools such as Julia, Python, and R not only makes the material far more accessible but also equips the readers of this book for their professional careers. I wish I had such a book when I was in grad school!
Viral Shah, Co-inventor of Julia programming language, Co-founder of Julia Computing, Co-author of Rebooting India
This important book fills a gap in the existing curriculum by providing a firm foundation in linear algebra, statistics, and coding for students who want to study advanced econometrics. And there's more: it also exposes students to methods of machine learning and computational statistics, offering a broader perspective on modern data analytic techniques.
Hal Varian, Chief Economist, GoogleA Primer in Econometric Theory presents key foundations and supplements them with good examples. It vividly shows the benefits given to us by decades of technical progress in econometrics and computational methods. Stachurski's writing style presents technical arguments in attractive and accessible ways.
Thomas J. Sargent, New York UniversityJohn Stachurski's text, A Primer in Econometric Theory is a concise and elegant book that provides a more conceptual introduction to econometrics that coincides well with my own preferred way of teaching the subject to first-year graduate students. It is clear, rigorous, and provides a large number of interesting exercises with solutions. I will use it in my own econometrics teaching and recommend it to complement other applied econometrics books.
John Rust, Gallagher Family Professor of Economics, Georgetown UniversityWhen I learned the fundamentals of linear algebra and economic theory as a graduate student, I found most textbooks and courses had an emphasis on theory but very little in terms of software. The emphasis on teaching these ideas through modern programming tools such as Julia, Python, and R not only makes the material far more accessible but also equips the readers of this book for their professional careers. I wish I had such a book when I was in grad school!
Viral Shah, Co-inventor of Julia programming language, Co-founder of Julia Computing, Co-author of Rebooting India