5
1
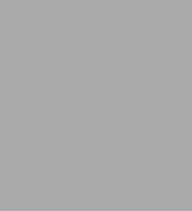
A Concise Introduction to Machine Learning
334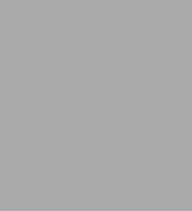
A Concise Introduction to Machine Learning
334eBook
$48.99
$64.95
Save 25%
Current price is $48.99, Original price is $64.95. You Save 25%.
Related collections and offers
48.99
In Stock
Product Details
ISBN-13: | 9781351204736 |
---|---|
Publisher: | CRC Press |
Publication date: | 08/01/2019 |
Series: | Chapman & Hall/CRC Machine Learning & Pattern Recognition |
Sold by: | Barnes & Noble |
Format: | eBook |
Pages: | 334 |
File size: | 14 MB |
Note: | This product may take a few minutes to download. |
About the Author
From the B&N Reads Blog